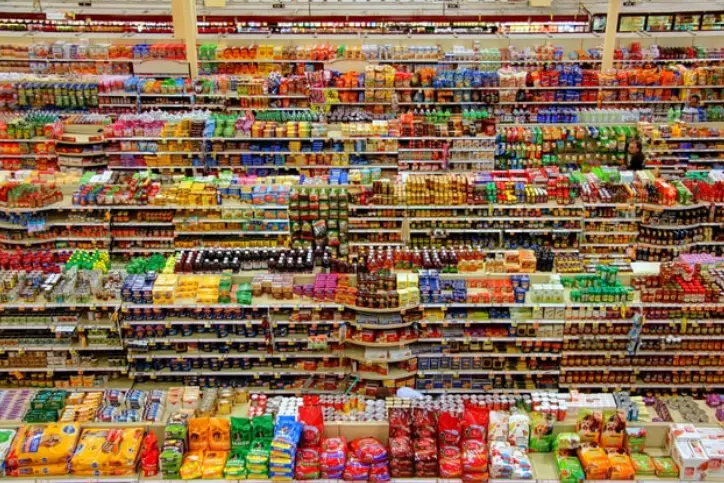
How AI Optimizes Demand Forecasting for Grocers
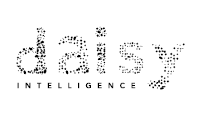
Grocers who rely on the discipline “Demand Forecasting” to live up to its moniker, i.e., accurately predict future demand for specific products, have, unfortunately needed to maintain a healthy tolerance for inaccuracy. In fact, grocery retailers today are challenged to properly manage and allocate inventory with forecasts having errors ranging from 40% - 60% plus.
The key word here is ‘Accurately’. Less-than-accurate demand forecasts lead to an “Overstock” situation, with too much inventory creating a drain on profitability. “Under-stocked” i.e., not enough inventory, means loss of potential revenue and dissatisfied customers.
It is important to note that there are already number of sophisticated demand forecasting software product suites available in the market. The problem is that their efficacy and potential has been limited by the available technology and computing capacity. They analyze every product SKU and transaction as if it were an isolated incident, and the problem with this approach is that it doesn’t reflect reality at all.
The enormous flaw with the way grocers execute demand forecasting is that it does not take into account variables in purchasing behavior such as product cannibalization, promotional cadence, seasonal impact and key product affinities, etc. because these occurrences are simply too esoteric or mathematically complex for standard demand forecasting tool-sets.
With Moore’s Law continually driving the cost of computing downward, it is now feasible to process all the data we collect. Further advancements in computing power will create an environment dominated by Artificial Intelligence (AI)
Successful demand forecasting requires a much more complicated formula than one can develop from simply running sales numbers for individual product SKUs. To predictably grow top-line sales and gross margin, a series of mathematical relationships that highlight and explain purchasing behaviors must be considered. Furthermore, external factors like competitive response to promotions and pricing as well as market demand have an impact on retailer performance. We call this the “ripple effect” and we use AI to measure it in an accurate manner. Key word again, Accurate.
With AI we have therefore unimaginable possibilities around demand forecasting. Before AI became widely available, just attempting to analyze billions of transaction records and correlating across a typical supermarket with 110k+ SKUs and trillions of product combinations was (quite literally) mathematically impossible.
AI enables us to achieve forecasting accuracies more than 10-15% over the standard which was developed around 20 years ago. For high-velocity products, AI can reduce the forecasting error rate to less than 5%.
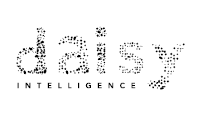