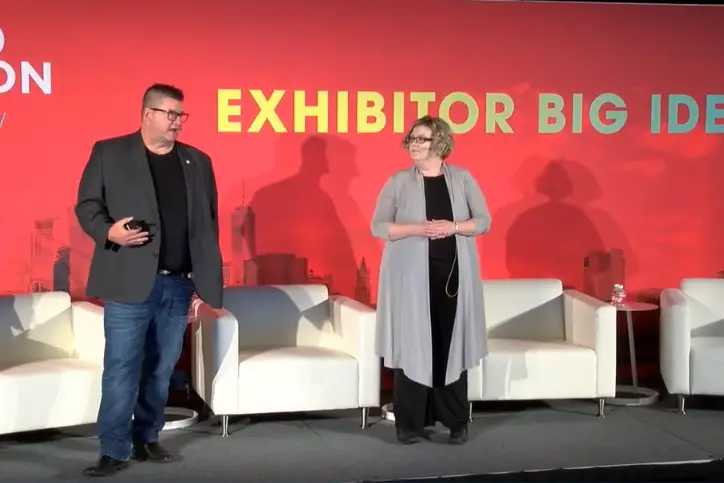
Big Show, Big Progress
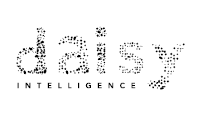
Year after year, NRF’s Big Show grows. It’s almost overwhelming how much there was to explore, learn, and share at this year’s event—all with 40,000 of our colleagues. One of the highlights of this year’s show was a breakout presentation from Daisy’s CEO Gary Saarenvirta and Alisha Tarrance, Merchandise Financial Planning Manager for SpartanNash. They presented on AI: The Challenges, benefits, and opportunities for retailers, and Tarrance shared insight on the positive experience SpartanNash has had on its AI journey since partnering with Daisy. She explained that much of the success has stemmed from the company’s buy-in, the adoption, and the commitment the retailer made to bringing “this know-it-all analyst,” as she joked, on board.
As in past years, the Daisy team spent three full days walking the Expo floor, meeting with customers new and old, and soaking up the expertise and knowledge the Big Show had to offer. And in doing so, we learned a few things about retail in 2020 and beyond. Here are three key takeaways from NRF Big Show 2020:
NRF Big Show takeaways
1. AI overload is real
The sheer breadth of technology available that a retailer can include in its business is almost overwhelming, from robots in the aisle to something as simple as e-coupons. And understanding that lends itself to just how challenging a retailer’s job is. AI is one of the solutions to these challenges, but more often than not what is being termed as AI isn’t really AI. Most of the AI on offer these days isn’t true AI, but rather, predictive analytics. For the retailer who is ready to talk AI, to know what they truly need, and how to discern the right solution for their organization without getting overwhelmed, they need to have a plan. They need to know where the pain points are in their business, and what you’re trying to solve for, and what business you’re trying to accomplish. You can’t window-shop for AI
2. The normalization of omnichannel
Three years ago, you wouldn’t have been able to walk five feet at any retail show without hearing the words “omnichannel” and “e-commerce” buzzing around. But that conversation has moved forward (and often now circulates around AI) as omnichannel and omnichannel solutions have been normalized into the CPG ecosystem. It is well understood that omnichannel and e-commerce are ubiquitous, a given in today’s retail environment. And it’s further understood that as a retailer, if at this point you do not have omnichannel as part of your strategy, you’re just not relevant in the retail landscape.
3. Partnerships are the strategy of the future
Retailers understand more than ever the role that technology is playing in their future success. While years ago, they needed convincing to work with technology partners—believing they could build the technology they needed themselves—that’s not the case anymore. Retailers have the data, but they need the solutions that will help them clean the data in order to take action. If there’s one thing retailers don’t need, it’s more data they can’t use, and because of this, they need—and want to—partner with companies that can deliver decisions, and cut through the mountains of data they already have to get to what they want to know:—what to promote, what to charge, and how much product you’ll need for any given promotion.
NRF 2020 Presentation: Daisy and SpartanNash
Gary Saarenvirta, founder and CEO of Daisy Intelligence, spoke at the session, “AI: The challenges, benefits, and opportunities for retailers” with Alisha Tarrance, Merchandise Financial Planning Manager, SpartanNash.
NRF Video Transcription
Gary:
Thanks very much for joining us on the last day of NRF and I'm sure you've been to a thousand talks…we got a decent-sized group here for this time slot. I'm Gary Saarenvirta. I'm the founder and CEO of Daisy Intelligence, and I'm here to tell our story and we have one of our customers here, Alisha Tarrance from SpartanNash, who's a user of the technology, and she’ll tell you what the reality of AI is all about - because she uses it every day. She'll tell you the good, bad, and ugly. There’s a vision, and then there's a reality story here too. We have a booth at number 637. So, feel free to follow up with us after.
So, the goal today is I'll define artificial intelligence. Because I think there's a lot of hype in the marketplace about what is AI, what isn't AI. So, I'll talk about that. And then, we'll talk about how we're applying AI in merchandising and pricing. Alisha will talk about the real-world experiences and how SpartanNash has implemented the Daisy system and go over the challenges and the outcomes that have happened there.
So, we'll start with what is AI. I think, for the most part, when people use the term artificial intelligence, they're really talking about statistics, or as I would say “statistics rebranded.” I'm an aerospace engineer. So for me, what NASA has been doing since the 1950s and what the US Air Force does, that's really AI. It's called Optimal Control. A human being didn't land the lander on the moon. Neil Armstrong didn't fly the lander. He told the lander, "This is what I want to do." And the computer figured out how to execute those flying instructions. If the computer on a fighter jet crashed, the pilot would eject immediately because the computer adjusts the flight controls a thousand times a second. So, I call that autonomous machine intelligence with human oversight. So, the human is the pilot asking the AI what it wants to do and the AI can execute the details. A simple example of autonomous machine intelligence is a thermostat. We know that if you add heat to an insulated box, it would get warm. If you add cold to an insulated box, it gets cold. You don't need a predictive model to do that. And, there's a sensor that measures the temperature and based on the difference between what you want and what the temperature is, the system very simply, autonomously decides whether to turn on hot or cold. So, that's autonomous machine intelligence in the most simple form.
In a more complex form, it might be an autonomous race car. So you don't drive a car with a statistical model. You wouldn't put a car with an empty brain on the road and let it learn on the fly and crash a million times and run people over. You would simulate what the physics of the car are. And fortunately, you have the laws of physics. You would simulate in a computer, a simulation of the world, and figure out what's the optimal way to drive a car. You could crash on the computer, no problem. We could do a hundred million hours of driving in one hour, drive more than all of humanity has ever driven. So, unsurprisingly, these types of autonomous systems like AlphaGo that beat the human goal player. They beat the human because they played more games than all of humanity has in all of history. So it's brute force computing that achieved and through trial and error, the race car logic, the AI decides what's the optimal sequence of steering, brake, and gas pedal to achieve the fastest lap. The reward is delayed from the instant. There is no instant reward - except that you're on the road. You have to sacrifice in the short term, slow down, for the corners to get the fastest lap. So, that's an autonomous machine intelligence. The historical data is only used to configure the simulation. What's the force of gravity? What's the friction of the tires on the road? So, you don't model the data. You don't learn from the data. You just configure the simulation and learn what the patterns are.
So, for us, that's what AI is. It's tried-and-true. You create a mathematical theory first, then you get the relevant data and you apply it. That's how the whole world was built. Not a single thing in your lives was built with a statistical model nor a sea hunt for data. So, I don't know why the world has turned this inside out. The scientific method is what's being used in the engineering world, and what we're trying to apply to business. So, again, what's AI? It's autonomous. There's no human in the loop. If 50 consultants show up with the statistical tool, that's not AI. So, it means no human in the loop, right? It's machine intelligence. So, I hate the term AI. It's computers. Computers do math better than people do since the beginning of computing. So, it's based on computer intelligence. It simulates alternative decisions. It says, "If I promote this product, what happens. If I don't promote this, what happens?" Right? Simulates alternatives more than people can, in order to learn what is the optimal set of decisions and it can learn new decisions that have never been done before, because you don't have to have historical data. We can find patterns and products that indicate that they're good, uh, products. And, there's a delayed reward. The goal is to optimize sales for over a year. So, to tie it back to the race car, a year of retail is like a lap around a race track, and how do you drive the retailer? You decide what's the weekly promotional product mix, what's the weekly pricing mix and what's the weekly inventory allocation. Those are the key inputs that you use to drive the retailer to get the maximum result. And overall, what's the role of the human? It's not to replace the person. The human is the pilot. The human sets the strategy. AI is not gonna tell you that you should be a high-low retailer or an EDLP retailer. It's not going to tell you whats your best category. It doesn't tell that. You set the strategy. The human is the AI's boss. So, we elevate the person. The person sets the goals and we as humans understood, we created the math. It's a human-driven system, not a random statistical system that just finds correlations and everything.
So, how disruptive this should be? It should be massively disruptive economically. What we've been able to do is grow promotional effectiveness by more than 100% which translates, on average, to about a three-to-five percent, year-over-year, same-store sales growth, massive, right? In the grocery industry, that would represent a 100% increase in total company net margin. If AI is the panacea, then these are the types of results you should be seeing. So, that's what AI is. These are some of the solutions that Daisy offers. I'll let Alisha introduce SpartanNash and stop hogging the stage here. Thanks, Alisha.
Alisha Tarrance: Thanks, Gary. As Gary indicated, I'm Alisha Tarrance. I'm from SpartanNash. I am actually a Category Planning Manager…or Merchandise Financial Planning Manager, depending on how many words you want to add in front of it. It pretty much means that I handle the promotions and the finances simultaneously for all of SpartanNash. We are a Fortune 400 company and the 5th largest distributor in the US, headquartered in Grand Rapids in Byron Center Michigan. What's funny about us is we're a distributor first, one that happens to do retail. And what we learn from our retail we can then give it to more than our 2,100 distributor partners. So, what we learn, helps us help them. We use our corporate-owned locations as little test packs and then we're able to help our independents, which is 2,100 strong, So, it's a really great relationship. We do have six different banners across multiple states. We do business in Michigan, North Dakota, South Dakota, Omaha, high-low values, uh, specialty. So, it can be very complex from our promotionally-driven planning model, which is why we love Daisy Intelligence.
So, why did we adopt it? Obviously, I just told you how complex our distribution network is and therefore, so is our retail ops. As many of you, I'm sure, in here are merchants, the days do not get any more hours…. but there always seems to be a lot more work that we try to press into those days for our teams. And, a lot of it is around making the right decisions for the right business at the right time, and it could be scary. There's a lot of risk-reward payoffs that happens with that. And, we needed a tool to help us. We can all run Excel reports. We can all take information out of MicroStrategy and dissect it a hundred ways to Sunday, but MicroStrategy never opened up and said, "Hey, you should probably do this, SpartanNash." MicroStrategy doesn't tend to talk to me even though I'm in it a lot. So, that doesn't happen. So, we needed something to help us with our decision-making and we haven't come across that. We needed something that could provide promotional insight with little risk, because, as we all know, our business is not getting any easier. It's a lot harder to get those increases in promos. The margins are tighter. The vendors are squeezing harder. Our independents wants more. Everybody wants more. The customer wants the lowest price. The company wants the highest return. Everybody is just squeezing everybody. So, we needed to find a different way to go to business and to go to market, so to speak, with our promotional strategy at a corporate level and we needed to understand that this wasn't going to be short-term. So much in merchandising, we will get “what happened this week.” All of the reports are run on a weekly basis. They run at the item level, category level. Week, week, week. Bucket, bucket, bucket. What did fresh do? What did this desk do? Everybody is bucketized and they're putting in their little round peg and so, this is what you need to do and here are the results. It happened because of it. So, we don't have a lot of time to actually measure what's going on, to actually see into the future, to see what we can do to be better and/or have, a lot of times, the ability to make drastic changes because it just means so much to the company if we make a bad decision. So, that was the biggest driving factor. We needed the tools to be able to hand over to our merchants, to be making the better decisions to grow our business in a world where it's hugely disruptive right now.
Gary: So, one of the things that we've learned is that merchandise planning is not a human task. So, if you have 50,000 products which should be, uh, in a large grocery store, and you have to pick 500 products every week to promote. You know, that 50,000 choose 500, that-- you want to-- that lot of math, that means there's 10 to the power of 3,600 possible combinations of products to consider, right? And, that's, that's a 10 with 3,600 zeroes. Well, there's only 10 to the power of 80 atoms in the universe. So, you can't do statistical modeling. I'll talk to you in a little bit about, it's the combination of products that matter because we know that products have a halo and cannibalization and pull forward or pantry loading, all those ripple effects. So, every week, the retailer does something you've never done before. Every week is a unique week. So, you don't have any historical examples. And if you've been in a business a hundred years, you have one data point a week because it's the combination, that unique combination. You have 5,000 data points out of 10 to the power of 3,600 possibilities. There's no way you can use statistical modeling or supervised learning to do this problem. So, that's why AI is not a human task. No human being could possibly look into that. It's no wonder retail is such a narrow margin business because it's so complex and astronomically difficult, and that's where computing can help.
So, how does Daisy work? We simply-- we learn some of the patterns. We configure our simulations from two years of historical TLog data. So, that's telling you 100% of what your customers are buying. We call that extreme personalization. We listen to what every customer tells you by their purchases. And, we don't learn from that data. We configure our simulation on it, and I'll talk about that in a minute. We use our Theory of Retail like the Laws of Physics. We create laws of retail. We configure our theory of retail using that TLog data to learn some of the patterns that are happening. Then, we simulate. We trade off all alternatives. Should I do product A or product B? Should I do milk or eggs on the front page? What if I did cheese? What if I swap out the cheese with bread? Try all those simulations. We do billions of simulations in a given week and then we deliver decisions to people like Alisha, who then looks out and goes, "What would Daisy say this week? What would-- what's going on the front page of the flyer? What should we put on the back page?" And the merchants and category managers and planners can then evaluate. They ask “Does this make sense? Do I believe it?” And you know, take away some of that, hard thinking that needs to be done. And then afterward, we measure what's the net promotional impact. What's the incremental impact to the business. Daisy AI is optimizing incrementality to grow the business.
Alisha: So, our journey has not been an easy one as Gary said. I don't think anything is and it's not inherently Daisy. It's really because we have to change the narrative of what we were doing at retail. So often what we do is, to what Gary had said before, it's easier just to repeat what you've done, because you know what the expected result is. It's a lot harder to say, "I think we want to try something out, and this is the result that we're going to get because of it."
Um, we did implement the product and price mix from Daisy and we effectively, by the way, she is a she. I would like to point that out. I let all my Daisy friends know that she's a she. She makes an incredible amount of decisions very quickly and she's very intuitive and smart and so she is a she. She has to be a she. There's no other way about it. So, and not only that, it actually was more fun to personalize her because if you ever sat in a meeting, um, if any of you are merchants you can raise your hand and have war rooms where you're like fighting for the front page and, "Oh my God. The poor girl has to be here. And no, but you know, I've got asparagus. And oh no, General Mills Cereal." I mean that's a constant battle. And so, it' really nice to sit in those and say, "Stop. Time out. Daisy indicates we should do this." And to have all the leaders in that room, "Who's Daisy? I've been here for 20 years and I have never met that employee. Who is she that's telling me how to run my paper." And so, it's really a conversation and it really makes the ingestion of the idea that a computer is helping us make decisions, a lot easier on the teams. So, you got to give her that personal touch and I recommend you do the same thing. It makes it-- it even makes it for a fun dinner conversation at the end of the day. It's hard though because we have to get her ingrained into our process and that can be the toughest thing, and it's tough not to measure something for a one-to-one relationship. I'm gonna put bananas on the front page because it does X. When Daisy looks at it, she's looking at our total store and how we're helping in the total store. She's breaking down the walls and saying, "I don't know anything but to be productive and I don't know anything but to help you grow and pick the right items as a store. Not as a center store, not as fresh, not as frozen, not as wine, not as anything. How can I help SpartanNash grow the business?" And she answers that question every time with as much intelligence as she can. She really helps guide us in those decision making.
Gary: How do we create the laws of retail? When you're creating the laws of retail, it's based on fundamental truths, truths that you know. We all know that products have a halo. For example, ground beef is a great product. All the grocers know that. Customers don't buy just raw ground beef except for my one crazy uncle who opened the [unintelligible] stick the fork in and it's just disgusting. But other than him, everybody who buys ground beef buys something else. If you're thinking, "Ah, Italian dinner," you're buying pasta, tomato sauce, cheese, bread. If you're thinking, "I'm going to make hamburgers." You're buying buns, condiments, produce. So, ground beef is a product that has a large use case. Consumers don't buy products. They buy solutions. And so, solutions that have multiple products in them, create a larger basket. Ground beef will create four or five more items for every customer and that's just them fulfilling their need. Contrast that to a case of water. The use case for water is buy the case of water, drink it. You don't need to buy other products to use the water. So that's the first thing. Think of products that drive a big halo. Don't promote the halo. Discount the ground beef, keep all the other items at full price. That's how you control margin. You don't have to give it away 'cause customers will buy those products anyways. But it's not all positive incrementality. Because the customer bought ground beef to make hamburgers, hotdog sales go down. You have to net out that negative effect. Because they bought hamburger buns and the halo of ground beef, that cannibalizes hotdog buns. They forward bought two weeks' supply because it was on sale. It was a great price. You pantry load it and put it in the freezer. So, those ripple effects are astronomical. If you have a hundred thousand products, you have 10 billion weekly ripples and some of these products ripple forward in time. That's way beyond human ability. And of course, we consider promotional elasticity, putting it on the front page will sell more than an inside page, doing a TV ad or a front of your website landing page, drives more sales. Some products have a longer life cycle than others, so that impacts how frequently you should promote them. We look at competitive pricing. What you buy at Christmas is different than what you buy in the summer. These are all common sense truths. Every one of you knows that and we've just assembled this into a mathematical theory that we can then simulate the impact of those product mix, price mix, and inventory allocation decisions to find what's truly incremental and will grow your business.
And then, we measure verifiably, what we call net promotional effect. We measure the baselines of everything Daisy measures. What would this product have done if you didn't promote it? That's the baseline sales. Then there's a promotional lift because you did the promotion. There was a full-price lift. You made a discount in pricing. You got some vendor funding and that resulted in the promotional lift that you created. That's incremental to the baseline. Most retailers stop there because beyond that is invisible. Then, Daisy goes into those billions of interactions, measures what's the incremental halo, the incremental associated sales. We know that that halo exists even for non-promoted products. So, we calculate what's the normal baseline you get. If you look at your data, you'll discover that the ratio of associated sales to item sales is the same every week, whether it's promoted or not. The recipe for a pasta dinner doesn't change because ground beef was on sale. So, you can get incrementality. If you sell 10% more ground beef, you'll sell 10% more pasta and you get that incremental halo. And then, we subtract cannibalization. You didn't buy hotdog buns, goes down a bit. You forward bought and pantry loaded a two-week supply. That's a negative impact. There's a price investment plus vendor funding. Add all those positives and negatives together, you get what we call net promotional effect which is an incremental measure of the impact on your business. We can measure stock-outs. There's a loss due to stock-outs. We didn't have the right inventory. We didn't make the right forecast. And then, we show what you left on the table 'cause you didn't listen to Daisy. Not to poke you in the eye but just to continue to cajole and remind and try to do that change management. Daisy doesn't know everything. Sometimes there's a storm went through and destroyed the farm field and blueberry prices went up. So, that's where planners like Alisha will go in the last minute. Say, "Let's make a change" or you got a great vendor deal, or you have access inventory you want to clear. Lots of good reasons not to use Daisy. We simulate. Here's a simulation example. If you simulate it, here's a price elasticity curve for ground beef. We would say, if you picked a price point there and you would choose a net promotional price there, that lowest price that drives 11,000 units, right? But now, if you look at every single product, we can simulate what happens when we look at all the products that are related to ground beef and we would choose a much different price than we've ever done in the past and double in that promotional effectiveness because we look at what's the impact of this price of ground beef on pasta, pasta sauce, taco shells. So, with the laws, the mathematical laws, we can simulate what you've never done to find solutions that drive incrementality. In this example, you know, we would double the net promotional impact of that promotion by considering that halo effect that occurs. So, maybe Alisha, you can talk about some of the reality and implementation challenges.
Alisha: As I had said before, nothing is ever perfect. And I've always said in working with various different software, it's very rarely the software's fault. It's generally the data that's either being put into it or the user error part, or user reluctance to accept it. And the-- it's always-- it's generally always been the same in my mind and Daisy is no different. Um, we do try to humanize her, like I said, it's a funny way but it's also intuitive because we have so many data pools and sometimes our teams, "Really? Another software? Another one? I have to do this?" And it's just, in their minds, you're adding another layer of complexity that's something that is very repetitious because of what we do every day in grocery. To them, it can sometimes be “You're adding this but I'm not seeing the immediate benefit because, again, it's not always about immediate gratification. This is about a long-term strategy for long-term growth. And so, if you're not measuring it on a daily, monthly, weekly basis, that's really going against the heart of a lot of what we do in old-school grocery retailing, right?” So, having that change mentality is really the hardest piece that we have every day and that is just a change management process that, um, we have to go through. We wanted to easily adapt her and quickly, to see how we could get her up and running, and, you know, have her talk to us. When your plan is far out as we typically are in a grocery, that can present some challenges of introducing some a new fork in the road so to speak.
So, we did it small. We did it incrementally and said, "Let's just focus on the small area and a small front page, and let's build that into our plans." And of course, it's all about changing process again and process change takes a long time in a big company. And then we-- it's all about the conversation and changing the narrative. We believe in Daisy and that starts at the top-down in our organization. Our leadership has rallied around it from the top-down. And so, if you get that kind of organic buy-in with all of your leadership, the narrative is always there. So, if you're at a meeting, the answer is always, "Well, I don't know. It's okay, let's go consult Daisy." She is like our little Wizard of Oz, or our Obi Wan so to speak, like "Oh, I don't know what to do. Okay, let's go ask Daisy."
When those narratives start to change, she becomes ingrained into your culture and it's made it a lot easier for us to do. We also, though because we are merchants and work in a business as we do, we have to align our KPIs, right? Some people's soft rewards are great and all but at the end of the day, the hard-defined goals have to be there as well. So, for the immediate users, which are business planners, we do recognize and align their strategic goals for their KPIs with the utilization of Daisy. And that's a work-in-progress as we work collaboratively with Gary and his team and how that looks, they report out to us on a weekly basis. So, we always have a measuring tool, a yardstick to see where we're at and it's easy to measure and it's easy for our team to understand our accountability and ownership into it, and that works really well for those, uh, merchants that still need that, "I need that hard goal." A lot of others just need the soft goal. They needed to be part of the culture and the conversation, and you got to make it easier. Not everything is as user-friendly especially when you're talking about AI and if you think of the sheer amount of SKUs that are in any grocery store, Daisy hasn't just all that. Our team as to help her and our team then have to sort of undigest that. So, both teams work extremely hard and then we both try to help each other to make it way easier at each other. And so, that's always, uh, an opportunity.
So, we are looking to save a lot of time. I wouldn't say we're quite there yet in our total roll-out but we also have only been doing these six months so it's not a long process and we haven't been on it very long. We haven't been dating her very long. Um, we're pretty much committed at this point so we're beyond the first date. Daisy is saving us time in our planning process because she spits out her recommendations and we listen. And that's what we do. And, our merchants get to spend more time on all of the other things that we are asking them to do. So aside from Daisy, they have to take care of customers. "Oh, by the way, put in your assortment. Oh, by the way, four stores called and they have a problem. The warehouse has a problem." So now, we freed up a lot of their time that was spent in planning because Daisy's done a lot of the thoughts for us. It's not all about just their intuition either. Um, we are trying to become a more data-driven organization, and so, it's the science with the art. It's the painter and the paintbrush. It's the neck and the head. However analogy you want to do with it. Merchant is not all science and it's not all art. It's really being able to find the blend of the two and making them work together to be successful and Daisy really represents that for SpartanNash at this time.
Gary: As what Daisy tries to do it's not about just the category, working category management, uh, where you treat every category like a silo and you run that category like a business. So, what we're doing is working with some of the leading category management thinkers like our great partner at TPG, The Partnering Group, some of the authors of that original paradigm. We're [inaudible] flipping the story from a vertical category view to a holistic horizontal view, looking at those interactions between categories. And given the competitive nature of retail ever more so today, it's always been competitive but even more so, you can't ignore those horizontal halo effects. So, that holistic view of promotional and total sales activity is important. So, it's what's best for the company, not one department or one category, and that's what the technology allows you to do. That's what is beyond human ability, to optimize total company sales and that, as Alisha has talked about poses a whole bunch of change, challenges around compensation, process, all of those things that need to be taken into account. Fundamentally, the technology works. If we can fly spaceships to Pluto, I think we can help retailers do a better job of pricing and product selection, right? But the change is the really difficult part. It's the human side of it, right? So, what's next for SpartanNash?
Alisha: So, for us, that really is what Gary said. One of our biggest opportunities is to take down those walls. Um, many of you know meat, seafood, center store grocery, frozen, we are very silo. Our planning and our processes camp in on a different timeline. I'm, you know, you could be talking with one vendor in a more of a UVC-driven environment, uh, more of a shelf CPG in grocery right now about a promotion we're going to be doing in August. If I went to the produce team and say, "Hey, let's play in your August promos using Daisy." They're going to laugh at me. And they do all the time, by the way. But that's finding a way to work within the confines of what we have as businesses. We have these niches. In produce, they need to work within the boundaries that they have because that's what keeps our produce fresh, that's what keeps everything working. Daisy also represents that, as what produce should we be doing and how can it help. And how can grocery support? Conversely, how can crash support grocery and we're tearing down those walls off. Somebody has to have two blocks, somebody has to have three. It's bakery this month. We're working at it in saying, "We are a company that has a strategy, and a mission, and a duty to our customers and our independents." What is the best thing for the company? And we are-- we're working towards that everyday. It's a challenge. It's hard to change a legacy system like promo overnight and that's just our objective, continually, every day. It's emails. It's how do we do this? What kind of this can we have? "Oh, by the way, have you done this?" And it's a lot of communication. Um, the scope-- we want to be 100% Daisy. I can tell you we're not quite there yet. It's actually because of the things that make SpartanNash unique that are also sort of, uh, holding us back from being a 100% Daisy utilization. Um, and there're some opportunities on our legacy systems and data that is stopping that as well but overall, I can tell you unequivocally, we are 100% utilized Daisy for all planning metrics for promos in SpartanNash. She is talked about at every promo meeting that we have. And we like it. Like I said, she's our little Obi Wan and we do not see that going away. We actually see that team work and camaraderie growing over the course of the next year, not only between the departments and the merchants but with our Daisy team and partners, and other partners we have in the business to fully integrate a solution that works for us. And that's also the other part that's awesome about Daisy is they listen, she listens. And it's nice to finally get a partner that listens.
Gary: So why now? Why is AI taking this high computing powers, really the fundamental resource? Moore's law has made computing astronomically powerful. The computer equipment Daisy has today, a million dollars of technology today, would have cost a billion dollars in 2003 when Daisy was founded. So Moore's Law in GPU computing enabled this massive computation. Now, look at those ripple effects wasn't feasible to do this 15 years ago and this is accessible to everybody. Our smallest customer has four stores. Some of our largest customers have thousands of stores like SpartanNash. So, this can work at any scale economically because the business case is growing year over year, same-store sales. That means you can afford that it's paid for out of those business returns.
So, you might say what's the big deal. We've been doing statistics for decades, you know. Regression was invented in 1805. Well, it's because of all of these different software products, you might have tools in forecasting, marketing, vendor management, they all do statistical models. All different math. Are they pulling the rope in the same direction? They're at odds with each other. They're optimizing different things. How do you know that vendor A and vendor B, they're not offsetting each other's gains because you're not modeling the business holistically? So, that's a key thing. Engineering systems, the space shuttle is not flown by 97 different disparate systems that don't talk to each other. You have to have all of these systems working together, pulling on the rope in the same direction, right? So, if you have one set of laws of retail, all of your software solutions should use that. With Daisy's goals should be the brain of retail to help you do what to do for things that are beyond human ability. Let's let people do what people are good at. Let the machines do what machines are good at. Machines do math and computing very complex problems. Human beings are great at strategy, innovation, inventing the math, setting the strategy. So, we believe that if you-- every retail decision was based on the theory of retail and an intelligent agent like Daisy, you could grow net margin by 200%, 300%, and you won't bank that money. Smart companies will reinvest that in price, reinvest that in innovation and overall, lower the cost of living for your consumers and have a massive impact on humanity. That's what I believe the game stakes of AI are.
I'm happy to answer any questions you have here. We have a few minutes left. We're also going to be at booth 637, just down the way here if you want to talk in person. Thank you for your time and attention, and thank you,
Alisha: Thank you for having me. Thanks guys.
Gary: Absolutely. This is a marriage between AI and people. It's not about AI replacing people. So, we're saying use the technology to augment human capability, not replace people, and I think, that's the use case for artificial intelligence. Not this hype that replace 40% of jobs. That's not what we should be doing. The goal is augment human capability by solving problems that people are not good at solving. Like the best customer service robot will never be as good as the best human customer service person. So, let's leave that alone. But figuring out the optimal price while we know that computers can do that better than people. So, focus on those problems first.
Alisha: It is good. It's the art and the science, the mix of the both.
Gary: Well, on TLog data, the transaction receipts are pristine. That's, you know, that's the money that runs the business and shows up in the finance system. Typically, promotional history data, some companies don't capture that well. So, promotion history is a bunch of work. We use some other technologies to scrape flyers and translate that and we build some capabilities to do that as well. Some of the product master data, you know, product hierarchy file, and things like price item groups for pricing typically need some work but that's what Daisy also does. She does some AI base data cleansing and data correction that's helpful. So, I mean, we have the technology we've learned over our customers that, uh, how to fix some of those data issues but the fundamental core data, TLog at every company is pristine and is the sales that roll up into your paycheques.
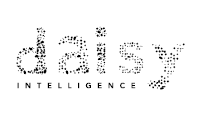