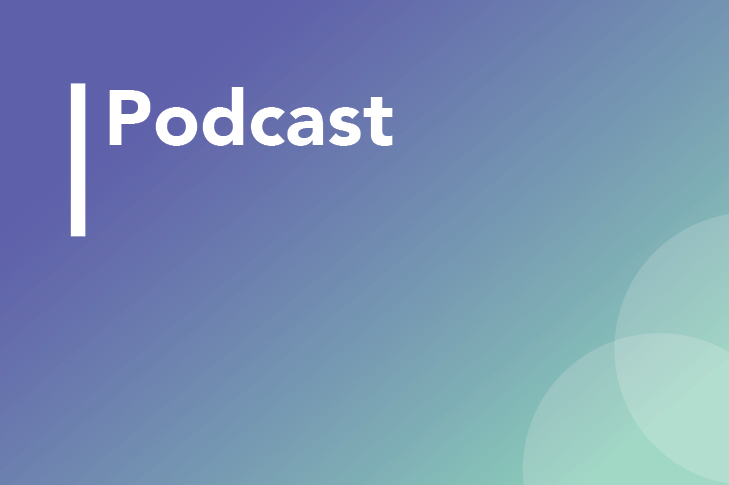
SuperDataScience Interview [Podcast]
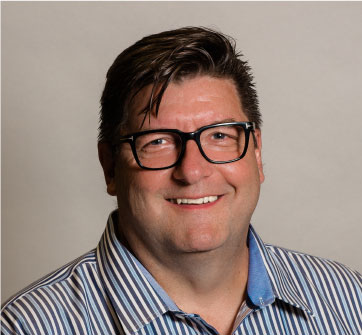
If you are here it is because you heard Gary Saarenvirta's interview with Kirill Eremenko on the SuperDataScience Podcast.
Gary is passionate about using AI to help large brands see what other's don't so you can drive higher sales and profits.
Listen to the Podcast
Podcast Transcript
About Gary
Kirill Eremenko: Welcome back to the SuperDataScience podcast ladies and gentlemen. Super excited to have you on the show. What an episode. What a conversation I literally just had with Gary Saarenvirta. This is a mind-blowing podcast.
Kirill Eremenko: Gary is the founder and CEO of a company called Daisy Intelligence, which does artificial intelligence, has a several platforms that provides artificial intelligence services to mostly retail and insurance and banking industries. And, some other companies such as in the healthcare space.
Kirill Eremenko: And what I found extremely fascinating about today's podcast is it was different from the commonly accepted perspectives. So, for instance, Gary says that you cannot learn everything from data. You have to start off with theory. And in this podcast, you will learn about the power of theories and how you can augment your theories with data or use data to find those features in the theories. So, it was just so interesting. I can't wait for everybody to check it out.
Kirill Eremenko: And the main thing what I loved about this podcast is it's different. And it's always good to get a different perspective. And this perspective is not just different in as a philosophically different perspective. Gary actually has results. They've worked with 30 plus billion-dollar companies in the retail space making hundreds of millions of dollars of extra revenue for those companies. They've worked with insurance companies and reduced false positive rates massively and at a massive value. So, he has actual numbers to prove what he's talking about.
Kirill Eremenko: And in a quick summary here's a couple of things that you will learn in addition to that refreshing perspective from this podcast. You'll learn about dangerous implicit assumptions. As we discussed, you'll learn about theory versus data. The power of theories. Toy problems versus real world data problems. Product interactions. Gary will talk about his theory of retail, his theory of insurance, even his theory of theories.
Kirill Eremenko: You'll learn about two types of decisions. We'll talk absolutely false positives. You'll learn about the spacial interaction model, traffic flow model, the concept of dividing the world in two and what humans should be doing, and what artificial intelligence should be doing. And the difference between artificial intelligence that leverages just data versus artificial intelligence that leverages theory and data, and what advantages that creates. And much more.
Kirill Eremenko: Very exciting podcast. I was literally listening to this with my mouth open half the time. So, make sure to check it out and enjoy the perspectives that Gary's about to share. So, without further ado I bring to you the Founder and CEO of Daisy Intelligence, Gary Saarenvirta.
Kirill Eremenko: Welcome to the SuperDataScience podcast ladies and gentlemen. Super excited to have you on the show because calling in from Toronto, we have a very special guest, Gary Saarenvirta. Gary, welcome to the SuperDataScience podcast. How are you today?
Gary Saarenvirta: Fantastic, Kirill. Thanks for having me on. I'm looking forward to chatting with you about artificial intelligence.
Kirill Eremenko: I'm looking forward to it as well. It's all my pleasure. And you mentioned stories. That you love to tell the story of how your company has grown and the work that you're doing. But even your own story is so interesting already. Like just before podcast I found out that you are originally Finland and now moved to Canada. Or moved to Canada when you were a child. How do you find combining the two cultures in your life? How does that affect your life?
Gary Saarenvirta: I mean, I grew up in a Finnish home. My parents raised us with Finnish cultural habits. So, I think that's really helped. Finnish people have a strong work ethic and the North American culture is a working culture. So, I had a lot of success with that. And so, yeah, I think integrating cultures ... Canada is a great place to live as well. Very diverse, and open, and accepting of all kinds of people so I think very easy to be from a different place in Toronto especially because everybody's from a different place here.
Gary Saarenvirta: So, it's great. I mean, I have the best of both worlds. Two great countries. Finland's always in the top five and Canada's usually in the top five places to live in the world. So, couldn't have asked for a better beginning.
Kirill Eremenko: That's awesome. Is it cold enough for you in Canada? Because Finnish people like the cold I hear.
Gary Saarenvirta: I love the cold and it's cold enough in the wintertime. But the summer's it's extremes. We might have minus 20 in the winter. But it could be plus 35 in the summer. So, I'm not a big fan of the heat and humidity. I prefer the cool. I keep joking with my wife that we're going to move to Iceland because we need to put a data center there somewhere. And she's like, "No, no, no." I thought that would be a nice halfway between the continents.
Kirill Eremenko: That's nice. In Canada, I actually don't know this, do you use Fahrenheit or Celsius for temperature?
Gary Saarenvirta: We use Celsius. I think the only two countries using imperial I think are United States. And there's one other one. I think the rest of the world has moved over to the international standard.
Kirill Eremenko: Yeah, in the UK they still use miles though. Not kilometers. But they do use Celsius.
Kirill Eremenko: Okay, well, very interesting. I'm very excited to have you on the show. I actually watched a couple of your videos yesterday where you're talking about artificial intelligence and reinforcement learning and giving some of those interviews. Very happy.
How Did Daisy Start?
Kirill Eremenko: So, I've got a lot of questions already. But start us off, could you give us a quick run down? What's your story? What is Daisy Intelligence? How did this all start?
Gary Saarenvirta: My background is in aerospace engineering. So, I have a master's degree in aerospace engineering. I went to the University of Toronto and studied computational fluid dynamics. And when I came out of school, my friend started a software company, so I joined them.
Gary Saarenvirta: Canada doesn't really have much of an aerospace industry, so I didn't want to move to the United States. So, we stayed in Canada and got involved in the software development and ran into some big companies.
Gary Saarenvirta: And I was really shocked at how little math and science large corporations used. And compared to what we did in engineering and science is it's all about math and science. So, I kind of accidentally walked into this career opportunity to try to bring math and science to business to help businesses operate smarter. And in doing so, they would increase the profitability.
Gary Saarenvirta: The goal is always to drive some kind of increase in sales and profits. And then I know that if a smart company makes more money, they don't bank it and give shareholders dividends. They'll reinvest it back in price and innovation, which impacts consumers.
Gary Saarenvirta: So, if you help companies be efficient, the cost of goods and services go down for you and I. And the cost of living goes down. And ultimately if you do that everywhere, you'll change the world. And that's kind of been my mission for the last 25 years. So that's how I started out getting into this kind of world of bring math and science to business.
Kirill Eremenko: Wow. That's a very cool perspective on how companies can actually help the world. And it's important to see it that way. I think any data scientist in that if you see it that way, you're actually doing the world a favor. Making a better place. That's very cool.
Kirill Eremenko: Okay, so there's 25 ...
Gary Saarenvirta: That's the way it should be.
Kirill Eremenko: ... 25 years ago you started this. And what was the mission when you started? To help businesses incorporate mathematics and data science AI in their work?
Gary Saarenvirta: Yeah, I first started doing predictive analytics. Statistical analysis. I think like a lot of young people today, I was amazed at how there's data correlations everywhere. And I was able to build statistical models to almost predict anything. And you could do that with, I thought, great accuracy.
Gary Saarenvirta: And I worked with a company in Canada that runs a large coalition loyalty program in retail. And we sort of did predictive analytics and were able to get what I thought were a lot of great results. Every model we did and every project we applied to ... direct marketing and likelihood for consumers to buy products, likelihood for people to respond to direct mail and direct email.
Gary Saarenvirta: And always got, we said, "Hey, we got 200% ROI, 300% ROI, 500% ROI. All these great results. But then looking at the companies that we were helping, looking at their annual reports, although we were seeing these amazing results from applying predictive analytics it didn't have an impact on the company annual reports at all.
Gary Saarenvirta: I'd begun to realize that the statistics is really insufficient. Predictive analytics doesn't really work to move the needle in business.
Why Statistical Models Fail
Kirill Eremenko: Sorry, I don't understand. How come If you're getting 500% ROI it's not having an impact?
Gary Saarenvirta: Because there's implicit assumption when you build a statistical model that there are no interactive effects.
You say, "I isolate this model." So, let's say I'm going to build a model to say which consumer is likely to respond to this product marketing campaign? But then you ignore that anybody who responds to that campaign that you assume there's no other interactive effects going on.
Gary Saarenvirta: So, there's no effect that happens over time. There's no effect that happens with other products. And that's an implicit assumption that you make. And that's actually wildly wrong, especially in retail where there's massive amounts of interaction.
Kirill Eremenko: Interesting. What's an example of an interaction that is not taken into account but does affect the end result?
Gary Saarenvirta: Yeah, so let's just say you were doing a promotion to promote Coca-Cola. And so, on an average week when you don't do a promotion you might, let's say, sell 100 cases of Coke. And then during the promotion you did a direct mail. You emailed consumers and you doubled Coke sales. And you go, "Great, look. We doubled sales. The ROI is massive."
Gary Saarenvirta: But then what you don't realize is that you also cannibalized other brands. So, Pepsi's sales and other cola sales go down. So, you need to net out those negative effects. So that's a displacement.
Gary Saarenvirta: And then you need to say, "Well, people forward Bob, they bought a two, three weeks supply of Coke. So, I stole sales from the future." And so, you net out all of those negative effects. You need to say did I really create incremental value?
Gary Saarenvirta: So, sometimes it does and sometimes it doesn't. But if you don't measure it on the balance of averages, the impact is actually zero.
Kirill Eremenko: Wow. That is such an interesting thing. I actually have never thought of it that way. That indeed isolated data science project, while successful, might overall for the business not bring as much value. And most likely in many cases probably brings less value overall than what you measure in the isolation of the project.
That's a very interesting thought.
Daisy’s Mathematical Model
Kirill Eremenko: So, what was your solution to this realization that you had?
Gary Saarenvirta: So, I went back to my engineering principles, right and started to say, if you're modeling let's say a car, you wouldn't model just one aspect of the car and just say, "I'm just going to look at the tires in isolation of everything else." You have to model the whole system. And that's what engineers do.
Gary Saarenvirta: So, we started to say let's model the whole company. So, build a mathematical model of the company. And I said then I realize that you couldn't learn that from data either. It's too complex. So, we started to build theoretical models like the physics approach. Take a fundamentals approach like the laws of physics.
Gary Saarenvirta: Einstein didn't come of the theory of relativity from data. He sat in a room and thought about it and came up with a theory. And then once he had the theory, he tested it on the real world. And so, that's the approach that science and engineering has applied for more than 100 years.
Gary Saarenvirta: So, I went back to my education and said, "Let's take that approach." And so, for retail we came up with a theory of retail. And for insurance and banking, a theory of risk. So, we create these theories that are a model of how the world works for that industry. And then we use that to solve problems. Where the mathematics is created independent of the data.
Kirill Eremenko: Very interesting. So that is actually a thought that probably opposes or contradicts what a lot of the guests say on this podcast. That data is the baseline and that's where we need to start. Everything comes from data. And it's really exciting to have a different perspective on the show.
Daisy’s Theoretical Approach and The Importance of Theories
Kirill Eremenko: So, can you tell us a bit more about this though? So rather than starting from the data ... I totally understand this example of Einstein. He didn't look at the data and then come up with the theory of relativity. The data for that didn't exist unless you looked for it. He actually sat down and came with this theory. And then went from there.
Kirill Eremenko: So, your approach is come up with a theory and then reinforce it with data, am I understanding this correct?
Gary Saarenvirta: Yeah. So, if you think of ... certainly Einstein had data. He had things like masses of the planets, and distance of the planets, and the force of gravity. And so, you use the historical data or experimental data to calculate features in your theory.
Gary Saarenvirta: So, let's say you couldn't drive a car with a statistical model. That's not the case. If you knew the location of every molecule in the universe ... knew its mass, position, velocity, acceleration and knew the history of all of that for all time, you could not learn the laws of physics from that data. The belief that you learn everything from data is wrong.
Gary Saarenvirta: And it feels like it's right because you find correlations everywhere. But correlations aren't causality. And you can find things like ice cream sales is correlated to murder rate. That doesn't mean you should stop selling ice cream.
Gary Saarenvirta: And there's correlations everywhere. It's like saying, "I could correlate the distance from the center of the earth to human population density and find that there's a correlation between distance from the earth and population. So, it's all clustered around the surface of the earth.
Gary Saarenvirta: Well, I don't need a statistical correlation to do that. I could just know that people live where there's oxygen to breath. And all the oxygen is on the surface of the earth. And it declines as you go up in altitude. And therefore, the population declines as the altitude increases.
Gary Saarenvirta: And that's a strong statistical correlation. But it's a fundamental theory says that people live where the oxygen is. And so that's the different approach.
Gary Saarenvirta: So, you find the fundamentals that explain the system you're modeling, and then create the math from the fundamentals, and then test it on the data. Here's my theory. Does it accurately predict the data and experimental results?
Gary Saarenvirta: And you can use the data to calculate features. Like in the case of retail the features we calculate from the data are price elasticity. We know that if you lower the price people will buy more. Promotional elasticity. If you advertise a product on the television, it'll sell more. We know seasonality, we know that consumers buy different products at Christmas than they do in the summertime. Or summertime in Canada because Christmas and summer are different here.
Gary Saarenvirta: We know that if customers buy a product, they buy halo products. If you buy ground beef to make an Italian dinner, you'll buy pasta and tomato sauce.
There's affinities. We know that one brand cannibalizes another. We know that there's forward buying.
Gary Saarenvirta: So, all of these fundamental things you can learn from the data, and you calculate those properties, and then you put them into the mathematical theory, and then solve the whole system.
Kirill Eremenko: Wow. Very clear. I actually got a good one for you. In the early 20th century, the creator of the P-test and P- values. I think is Ronald Fisher. We all know him as Fisher. He was actually using P-values to prove that not smoking causes lung cancer, but lung cancer causes smoking. Speaking of correlations and causality. It was interesting.
Kirill Eremenko: As you say, you cannot learn everything from the data. You need some sort of theory behind it. And some sort of understanding of how the world works to augment your data explorations.
The Advantage of Combining Theory and Data and The Game Tree
Kirill Eremenko: That's a very interesting approach. How does combining theory with the power of data, like using the two rather than just one, what kind of advantage does that give you in your industry?
Gary Saarenvirta: Well, we calculate the features from the data. So, you need the data to calculate properties of the world. Like I said those things like price elasticity, promotion elasticity. So, you use the data to calculate real facts about the world. So, it's based as a fundamentals approach.
Gary Saarenvirta: You can only really model toy systems with data. And you can learn simple systems from real interaction with the world. So, a simple system would be the game of Go. So, in any given move a player has maybe less than 100 possible moves they could make. So, the number of actions is small.
Gary Saarenvirta: The game tree is infinite but at any moment the number of moves is small. And you know how the world perfectly works because there's a game of rules that are perfect.
Gary Saarenvirta: So, if you put two computers to play against each other you can randomly play and find that you play a 100 million games in an hour if you have enough computing power. And you can find sequences of moves that have never been played before. You can play more games than all of humankind has ever played with a computer.
Gary Saarenvirta: And then is it surprising that we would find better ways of playing? If the average person in a lifetime plays Go for 10,000 hours and you play a computer that played for 100 million hours, is it a surprise that you lose to the computer?
Gary Saarenvirta: So, I think there's this overexciting thing, "Oh my god. It was a major breakthrough." We did a hack-a-thon at the University of Toronto where we played ultimate tic- tac-toe. I don't know if you're familiar with that game.
Kirill Eremenko: No, not really.
Gary Saarenvirta: You have a tic-tac-toe game in every corner of the tic- tac-toe board. So, in every box there's a ... And to win that outer box, you have to win the inner game.
Gary Saarenvirta: So, the game tree is infinite. We played with a bunch of university students. We opened it up at the University of Toronto. And we created a random player that did 500 milliseconds of random search on a GPU card. And just randomly made moves and played random sequences to the end of the game.
Gary Saarenvirta: And so, it's a completely random player. And then that played against the best ideas that students would come up with. So they came up with their best logic and strategies, and the best player who won the tournament ... we had a head to head tournament with absolutely 70 teams ... and the student team that won didn't lose a single game all tournament long. And then they played the Daisy Random Bot and we beat the student 100 games to nothing. With 500 milliseconds of random search.
Gary Saarenvirta: So, it's just showing that in toy problems it's not surprising that you could beat the best that a human can come up.
Kirill Eremenko: So, just to clarify. So, tic-tac-toe is the game with the 3x3 box, and you put the X's and the O's inside, correct?
Gary Saarenvirta: Yeah. If you search ultimate tic-tac-toe you can see the rules where there's tic-tac-toe game in every square of the outer game. And to win the outer game you have to win the inner games inside each square.
Kirill Eremenko: Got you. And when you say basically random, does it mean that the AI was working at random? That means you gave it 500 milliseconds. So, what that's half a second to do a random search. Basically, do learning on how to go through lots of different iterations. Come up with a policy on how to play the game within half a second.
Gary Saarenvirta: Yeah, I came up with a random policy though. It decided on it's next move randomly. So, the policy was just making a random move and assume the opposition player makes a random move, and then play out the sequence of games. And then find out which first move had the highest probability of a win when you played randomly.
Gary Saarenvirta: So, it was just purely random. So, there was like zero intelligence to it. And we beat that a smart engineering student ... some of them graduate students ... could come up with in 24 hours. And we called it Random Bot just because we wanted to test the game. We weren't actually intending to us it to play.
Gary Saarenvirta: But then at the end of the tournament we thought, "I wonder how a random bot's going to do against the best that the humans could come up with. Because we gave the students GPU’s, but it was too much for them in 24 hours to do that. So, our random bot killed.
Gary Saarenvirta: The point is that to me says that it's not that the brute force computation beats a human, it's not surprising. So, the game of Go and the chess when you do reinforcement learning, it is an accomplishment absolutely. But it's not this singularity type accomplishment that people think. There's very little intelligence in it. It's brute force computing.
Kirill Eremenko: Okay. Got you. So, it would be very hard to transfer something. I think that’s actually what DeepMind, the company that created AlphaGo and AlphaZero, what they're faced with right now. They are finding that it's extremely hard to transfer those results, which are essentially research results, into real world solutions. Like solutions for businesses, for making products, making the world a better place.
Kirill Eremenko: In fact, I think DeepMind in 2017, I think it was, they made a net loss of like $350 million or something like that at the bottom line. Simply because most of what they're doing is mostly research. Which in many cases does not really translate into useful products or solutions?
Kirill Eremenko: So, would you agree with that that it's ...
Gary Saarenvirta: Yeah, absolutely. Because a game like Go, or Chess, or even driving a car, the number of actions you take are so small you can learn in simulation. And in the case of a car, you have a very good simulation. The laws of classical mechanics. And the games, you have rules of the game. So, those are toy systems and you can actually learn the system can learn in real time playing against each other. You play the simulation faster than time so you can learn quickly.
The Game of Retail and The Game of Go
Gary Saarenvirta: But if you look like the game of retail, let's say. So, if you agree with the concept that customers don't buy products. Customers buy a use case or a solution. So, you're buying a meal. I'm buying an Italian dinner; I'm going to buy five products. So, I'm buying a solution to my desire to make a meal, right.
Gary Saarenvirta: And you know that there's these interactions. So, if you buy in the fact that products interact then if you have 50,000 products to choose from and you have to promote 500 products a week for a month. 50,000 choose 2,000 approximately let's say. That's 10 to the power of 3,600 combinations to choose from.
Gary Saarenvirta: Well, 10 to the 3,600 is more than the number of atoms in the universe. You can't do this with labeled. There's not enough molecules to create the labels. You can't learn that from interacting with the world because there's too many action states. So that's where you need to build a simulation of that system and then search that space. And that's where you come up a mathematical theory first.
Gary Saarenvirta: So, the fact that deep learning, doing deep reinforcement learning or deep learning to learn simple supervised learning problems, they apply to very toy situations and real-world systems, for the most part ... especially commercial businesses ... are infinitely more complex than the game of Go.
Gary Saarenvirta: The game of Go is a game tree of 10 to the power of 200 or something like that. Retail I just showed you picking products is 10 to the power of 3,600. So that's more than 1,000 orders of magnitude more complex. That's the real world. And so, you can't learn the theory from the data. You can't learn it from interacting with the world in real time. That's why you need to create this human created theory like Einstein did.
Advice for Building a Career in Data Science
Kirill Eremenko: Okay. So, what does somebody do? We have a lot of listeners who are building a career in data science. What is your advice for them? Is it to stop learning reinforcement learning?
Gary Saarenvirta: No, I think you need to measure the actual impact of what you're doing on the toy ... If you're in a business and the goal is to make money. Does it actually create money? Does A, B testing, control cell testing? That's bogus unless you measure all the interactive effects.
Gary Saarenvirta: And, there are some problems you could solve with data. But make sure that you're not ignoring important interactions. That you're not making implicit assumptions that are wrong. So, make sure that you innumerate all those assumptions and then see, "Can I still learn this problem from data?" I think that's an important thing.
Gary Saarenvirta: Really look at the causal theory approach. What are the fundamental factors that explain this? If you look at economics. Economists create a mathematical theory and then try to apply the theory. It's an approach that's been used that we seem to have forgotten about.
Gary Saarenvirta: The entire world around us, from the chair where both of us are sitting in now, somebody engineered that. They didn't use statistical analysis to design your chair. They used the laws of physics. And so, that's worked for over a 100 years. Why have we stopped doing that? It's because data is everywhere, and we think it's the panacea, and I think the world will come to a screeching halt shortly and say, "You know what? Doesn't actually work."
Kirill Eremenko: Very refreshing. I've actually fallen into that pattern myself of saying data is the panacea and data is everything. But it's really great to hear that that may not be the case.
The Laws of Retail
Kirill Eremenko: Okay, so laws of physics to design a chair or drive a car. Laws of biology and chemistry to design drugs, and medicine, and pharmaceuticals. What's laws for business like for instance retail you mentioned a few times?
Gary Saarenvirta: Yeah, so in retail for example, let's say bricks and mortar retail. It matters where your stores are. So, putting your stores near where the people live so you could optimally design the optimal location for your stores based on the population and where your competitor's stores are. So, there's a mathematical theory there and you can open up any textbook in the geography department, spatial interaction modeling that's called. And they have mathematical theories on how to do store location.
Gary Saarenvirta: Combine that with when people walk inside of a store if you put the products at eye level in a shelf that they walk by, they're more likely to buy things at eye level than above eye level or at the floor. So, there's a theory of planogramming based on the traffic patterns of consumers walking and where the products are.
Gary Saarenvirta: You can come up with a simple traffic flow intercept model based on proximity of the number of items on the shelf as a percentage of the total number of items. Can I find the product? Simple traffic flow intercept model that exists. And then looking at all these interactions, the halos.
Gary Saarenvirta: So, we've assembled mathematical equations from those three things and put them all together. But people won't go by competitors stores to save $.10 on carrots. They're not going to ... People will fly across the world to get a free gold bar. Location matters. So, all of these are the fundamental concepts.
Gary Saarenvirta: And then we design a theory, mathematical equations that encapsulate those. And it's not like it's a blank slate. Open up a textbook and you can see there's people doing this today. Spatial interaction modeling, traffic flow modeling ... which is the intercept model, which is customers walk in stores.
Gary Saarenvirta: So, we just put together things that have been done and that's the way the world works. You build incrementally on what's out there.
Why Focus on Insurance and Retail?
Kirill Eremenko: Fantastic. You mentioned retail and in fact, Daisy Intelligence works predominantly, as I understand, in retail and insurance or insurance plus banking. Why did you pick those industries?
Gary Saarenvirta: They're historically the data rich industries. We have one healthcare client as well. So retail, banking, insurance, telecommunications, manufacturing, government. These are the data rich industries. Which means that they're super complex business processes. And making decisions about these business processes is beyond human capability.
Gary Saarenvirta: It's beyond human capability to pick the optimal combination of products to put in a grocery flyer. And the humans that do that today when we measure the effectiveness of their product selections, it has very little correlation to total store outcomes.
Gary Saarenvirta: And so, we're applying machine intelligence to problems that are beyond human capability. That's the idea. And then let people do what people are good at, which is ambiguous thinking, interacting with other people. Let's free people up from these nearly impossible, highly repetitive, complex, occurring volumes of millions of decisions a day. Let the machines do that. Let people do what people are good at.
Gary Saarenvirta: And so, that's why retail has a huge volume of data. Banking, insurance huge volume of data. And there's complex interactive effects that take place. So that's why we pick those. And then healthcare, obviously that's another interesting industry as well.
Daisy’s Results in Insurance and Retail
Kirill Eremenko: Okay. And what kind of results are you seeing with these industries?
Gary Saarenvirta: In retail it's insane. So, we've been able to grow total company sales by we've seen on an average of 3% with kind of half change management complete. The human change management is the big challenge. But with about 40% of our decisions being implemented, we've been getting 3% sales. We have comp store sales, which is how retailers measure how did this store do this year versus last year.
Gary Saarenvirta: We've done that for companies with sales of over $30 billion. So, we've grown their sales by $1.5 billion. And retail typically is a 1% net margin business grocery retail. So growing sales 3% means we doubled their net income. Open the annual report, go to the very bottom line on the income statement. And if that number last year was $100 million, then this year it'll be $200 million. That's what the result we've had. Because it's common-sense. If you build a theory base on the fundamentals, it works surprisingly.
Kirill Eremenko: Wow, that's insane. When you say 3% it doesn't sound as impressive. But when you say you grew sales by 3% of a $30 billion business. And then also if you look at the net income, basically that flows down into doubling their net income from $100 million to $200 million as an example, that is insane. Those are amazing results.
Gary Saarenvirta: And if you go look at some of the other statistics, vendors, their value statements don't make sense. When you say, "I grew sales 10%." Well, if you grew sales 10% of the whole company, you'd have like quadrupled their profit. You would say, "I grew profits by 400%.
Gary Saarenvirta: But because you're not saying that, that means you did an A, B test, you grew this category by 10% compared to what you did last week, or last month, or in market A versus market B. You ignored all the interaction effects and maybe nothing happened. Maybe something happened but you don't know because you didn't measure that.
How Daisy Works With New Customers
Kirill Eremenko: Okay. So, tell me then, when a new retail company comes to you and says, "Gary, would you be able to work with us?" What is your first step to do the due diligence? What are the steps that you take? How do you assess whether or not you can help a specific client? What are the requirements?
Kirill Eremenko: I'm just curious of how you think as a consultant or somebody who's about to go in and make massive changes to the businesses profitability?
Gary Saarenvirta: So, we have a product. We sell a shrink-wrapped product. There's no human in a loop in our product. And ultimately in the long run, there's no human in the loop on the client's side. I think retail will ultimately be retail planning. A lot of it will be automated and done autonomously.
Gary Saarenvirta: So, we go into the client. What we've learned having done about 25 retail clients is that we need to assess the client's willingness to change. If you believe that the future of retail is not the same it is today and you're ready to change, and you want to change, that's the first test. Because if you're not willing to change the way you do things ...
Gary Saarenvirta: If you don't believe that a machine could do better than your people, then we're going to have an uphill battle. And so, we're lately starting to qualify and ask that question. "Will you trust a machine to make seriously important decisions for your business?" And if you're not willing to give that a shot then we're going to have an uphill battle. And so, we'll at that moment stop selling to that customer.
Gary Saarenvirta: Once they say, "Yeah, we're all in. We want to be a retailer of the future. We want to move in the direction like Amazon does today." Then great. Then we take historical data. We take as many years of historical transaction data that has every single transaction in bricks and mortar, and online, and that historical data. Because from that we learn all of the features of our theory. All the things I've talked about ... halo and elasticity. So, we need all the history to do that.
Gary Saarenvirta: To get seasonality you need multiple years of data to see seasonal patterns. And then you'll also have all these external effects in that data. The external how the competitive effects, macroeconomic effects. So, having a long history of data gets us all of that and allows us to calculate all these features that are kind of on average with respect to external impacts.
Gary Saarenvirta: And then from that we have a theory. We test our theory against the client’s data. We go, "Look, it works." And so far it's worked at every single client that we've done it at because it's based on fundamentals. And it takes us about a month to initialize the system. And then we start delivering decisions.
Gary Saarenvirta: We say, "Hey, next week you should promote these 100 products and here's the prices." And then the clients will start to execute it, test it. We'll measure the impact, show them the financial results. It takes typically three to six months to start to see financial results. And then we fight this change management battle to get them to buy into it.
Gary Saarenvirta: And then maybe change the role of the merchant in the future so the people who do this for a living, half of their job should be given to a computer and they can focus on the other half of their job. That's the challenge.
About the Daisy Platform
Kirill Eremenko: Okay. So, going in and getting all this data from the client allows you to tailor your platform. It's a platform that you offer? Not just a consulting service?
Gary Saarenvirta: It's a platform, yeah. It's a prebuilt platform. We're not a consulting shop. We're a SaaS company. The product is built we just feed the data in. That's the only part because every customer's ... the properties of the data.
Gary Saarenvirta: So, it's like the force of the gravity is different on every planet. So, every client is like another planet. So, we use the data to measure the mass of the planet, the force of gravity. Is it the same universe? We measure halo, cannibalization, seasonality ... those all vary slightly from client to client. And so, the historical data lets us calculate those features that we then put into our laws of retail.
Kirill Eremenko: Yeah, laws of retail. I love it.
The Challenge of Change Management
Kirill Eremenko: I'm a bit surprised that you with such a powerful system have, you mentioned I think, only 25 retail clients. I'm assuming these are large players that you've handpicked, selected. But have you thought of making this a self-serve platform where any retail store could come onto your website and say, "Hey, I want to subscribe to this," and then feed in their data themselves? What is the gap there? Is there a gap of knowledge, a gap of commitment from customers that they won't be able to feed in the data themselves?
Gary Saarenvirta: Yeah, it's a system. I've learned that customers, clients can't manage very complex systems. And so, this is a super complex system. So, we manage the system for them and help them with the recommendations.
Gary Saarenvirta: The challenge is the change management. And so, we're growing. We've triple, triple, doubled in the last three years as a company. We plan to double double. Our goal is to be the next Google, next Apple.
Gary Saarenvirta: I don't want to sell the company. I want the Daisy brand to be up there. Because I know if I achieve those heights then we'll have changed the world for the better. That's the company mission.
Daisy and the Gartner Award
Kirill Eremenko: That's awesome. Congrats. Last year you got, I see on your website, the Gartner Award for Cool Vendor for 2018. That's very cool.
Gary Saarenvirta: Yeah, we got recognized Cool Vendor. So, they're seeing us as up and coming. So, people don't know we exist so we're getting a lot of interest in the company based on the results. And it's challenging for retailers, you know, the retail employees to say, "Hey, a machine can pick products better than a human can." And I'd say, "Well, it's not that surprising. We can put men on the moon, and we can fly spaceships to Pluto. I think we can pick optimal prices or optimal product combinations. I'd say it's not a stretch." So, we're bringing NASA like technology to business.
Kirill Eremenko: Yeah, with your aerospace background, right?
Gary Saarenvirta: Yeah.
Kirill Eremenko: That's awesome. Wow, fantastic.
How the Daisy Platform is Implemented in Insurance
Kirill Eremenko: We'll, we talked a lot about retail, and I think this is amazing and I'm really happy to have you on the podcast at this time before this goes huge and with that kind of growth, it's just a matter of time. If you're doubling and tripling your business every year, it's just a matter of a couple of years before you hit that exponential kink and then you're just up there.
Kirill Eremenko: And what that means why I'm happy is because for the sake of our listeners, if anybody's in retail, if you're a business owner or executive, or you know any executive I have a strong sense that this could be a big game changer. And this would be a great time to jump early onboard.
Gary Saarenvirta: Absolutely. Give us a call if you're a retailer. If you're an insurance company, we can help you eliminate fraud. And then ...
Kirill Eremenko: Let's talk about insurance. We talked about retail. What do you do in insurance because it's such a different world to retail?
Gary Saarenvirta: There's two types of human decision making. One is: What are the inputs that get the desired outcome? So, what decisions do I need to make that result in the best outcome? So that's kind of what we're doing in retail. And there's the laws of retail in the middle. Like put these inputs into this system and I get this output. And then I try to optimize that.
Gary Saarenvirta: And, then another type of decision is: Is this different than the normal? So, when you go to a doctor, the doctor takes your blood test and goes, "Oh, these readings are different. You're sick." So being an outlier means you're sick. You could be a high-risk credit lender. Fraud is an outlier. Crossing the border could be an outlier, which might mean you're a terrorist, tax audit outlier.
Gary Saarenvirta: So, those are the two types of decision making. Are you different than the norm? And what inputs get the maximum outputs? And in fact, every business is a combination of those two things.
Gary Saarenvirta: Some businesses are more of this interaction effect versus outliers. And some businesses are more outliers versus interactions. And so, in insurance we identify what are the fraudulent transactions or claims? Who are the fraudulent people and who are the fraudulent networks of people? And we identify those.
Gary Saarenvirta: And, you can't do that with predictive analytics. That's a misnomer. And I see a lot of false statements about ability of prediction to identify fraud or other rare events.
Kirill Eremenko: Okay. So again, you created a platform, I'm assuming, which uses data.
Gary Saarenvirta: Yep, a platform. Yep, we have a theory of risk that we create. We've filed patents on our theory of retail stuff. We're filing patents on a theory of risk. We're filing patents on how to create theories for business. So, you can stay tuned to those things.
Kirill Eremenko: The theory of theories. I love it.
Why Predictive Analytics Does Not Work in Insurance
Gary Saarenvirta: Because there's a way to do this. And then in insurance the idea of predictive analytics being used to identify fraud, and I'll talk about that a little bit because it's important because of the mis-hype out there.
Gary Saarenvirta: So, let's say you built a deep learning model to identify fraud that was 90% accurate. And we see claims that are 95% accurate. So, let's say 90% for round numbers. It was 90% accurate detecting at fraud. And let's say you have a million claims. And of those claims 1% is fraud. So, of the million 1% ... or 10,000 ... is fraudulent.
Gary Saarenvirta: Because 90% accurate I got 9,000 of the 10,000 correct, right?
Kirill Eremenko: Mm-hmm (affirmative).
Gary Saarenvirta: So, I mean I got 1,000 wrong. But in the 900,090 that weren't fraud, I also got 10% wrong there. So, I got 99,000 wrong in the not fraud.
Kirill Eremenko: The false positives.
Gary Saarenvirta: So actually, I got 9,000 right and 100,000 wrong. So, my accuracy's not 90% it's actually 8%. In fact, when you're predicting rare events, you have a massive false positive rate. And that's okay if you're doing direct mail because the cost of getting it wrong in an email marketing campaign, who cares? You're just carpet bombing the world.
Gary Saarenvirta: But if you're wasting money on doing investigation, go to YouTube and look up false positive breaking events in autonomous cars. I won't pick any brand of car because I don't want to crap on anybody. But look at false positive breaking events in cars. And you'll find people who drive kind of these electric cars with the driving aids that there's a lot of false positives.
Gary Saarenvirta: And, you'll find videos of cars not even stopping while they plow into a stationary target. Because there's so many false positives that come up in the breaking systems that the manufacturer dials those systems way back. Because it's not a prediction problem. It's a physics problem.
Gary Saarenvirta: And so, any model predicting rare events, and guess what, any event worth predicting is rare, which is basically every event. Like image detection. You do images of cats versus not cats. Well, if I was driving a car, what percentage of images would have a cat in them? A tiny fraction. Even less than 1%. So it would be even worse than the math I quoted to you before.
Gary Saarenvirta: I'm 97% accurate at predicting a .01% thing which means my false positive rates 99%. So, it means I'm actually terrible at predicting a cat.
Kirill Eremenko: Wow. That's such an interesting perspective. And so, how does your product differ in that sense?
Daisy’s Different Approach to Insurance
Gary Saarenvirta: We take a different approach. We look for outliers in fraud. So, it's a rare event. But we're looking at what is the outlying behavior? Looking at all the attributes about a claim, about people, about networks of people and say, "Is this claim," we compare this claim to every claim we've ever seen before. So, if you have 100 million claims a year, we look at 10 years of claims.
You say, "How does this claim stack up to the previous billion I've seen? How does it stack up to claims that occurred in Toronto versus claims in other cities? How about claims for a car accident of this brand of car in the wintertime? Does that look normal with respect to a claim?
Gary Saarenvirta: And, the more peer groups that you compare the claim to you'll find it's an outlier. So, if I had 1,000 attributes that I'm comparing to and each of them that was only 1% accurate. So, it's 99% wrong. .99 to the power of 1,000 is 0, which means that if I'm monitoring outlying behavior on 1,000 attributes, there's a 0% probability that I will get it wrong at identifying it as an outlier if I look at the total set.
Gary Saarenvirta: And so, we use very sophisticated math to do outlier detection because statistical outlier detection doesn't work because everything's an outlier in high dimensional data.
Gary Saarenvirta: So, we use type one fuzzy logic as a method. And, we'd use some prediction and rules, but we use the output of predictive models in a completely different way, which I don't want to talk about yet because I haven't filed the patent.
Kirill Eremenko: Makes sense.
Gary Saarenvirta: So, call me in six months and I'll tell you about exactly how we do that. But it's combining every technique. Rules, prediction, peer analysis and social networking. And, if a person is fraudulent and all four of those methods agree, then the likelihood of it being a false positive is slim.
Gary Saarenvirta: So, using every mathematical method possible, if all the methods agree that there's something odd going on, then the false positive rate is lower.
Daisy’s Results in Insurance
Kirill Eremenko: Okay. And what kind of results does this allow you to bring to your clients?
Gary Saarenvirta: So, on average we see in most insurance companies anywhere between in healthcare insurance ... which we've worked a lot in ... we think 15% to 30% of claims have some element of fraud or abuse. So, we found millions of dollars of claims that haven't been found before.
Gary Saarenvirta: The challenge in insurance is that there's human investigators that look at every fraud alert. So, we're trying to convince our clients and say, "Hey, you need to let the machine make the complete decision and don't put a human in the middle."
Gary Saarenvirta: So, some of our clients can look at 100 fraud alerts a month. And when we can find 100 alerts a millisecond, so we need to let the machine make some of the decisions. So, we're working with our clients to say, “Let the autonomous machine make the decision on whether we should process this or not.” Take the human out of the loop and then we'll find that in a $1 billion business you'll find $100 million of fraud and abuse, and we want to eliminate that.
Gary Saarenvirta: So, that's the challenge. And the change management is: Human beings look at every fraud alert and every risk alert. And we need to let the machine do it so there has to be that change again. Do you trust the machine to run a significant part of your business? And, businesses aren't there yet. That's the huge world change that's going on right now.
Will AI Replace Humans?
Kirill Eremenko: Wow. I'm just finding myself listening to this literally with my mouth open. This is such fascinating stuff. So, retail and insurance ... sounds like you've got those two covered. Interestingly you mentioned that you want to take the human out of the loop. And in one of your online videos I heard you answering the question, "Will AI replace humans?" I found it very interesting. Could you also share your thoughts here?
Kirill Eremenko: If you take a human out of the loop in retail, in insurance, and in menial industries, what kind of future are we looking at?
Gary Saarenvirta: I think it will just change the job role. It won't eliminate the role of the person. So, I'd say divide the world in two. Use computers to solve problems that are beyond human capability. So that means we're helping humans. And then let people do what people are good at. Strategy, human interaction, creativity.
Gary Saarenvirta: And, we shouldn't apply technology to replace people. So, I say we're going to change the role of the person. But you can't let the machine intelligence on its own, you still need to have human oversight. And the human beings will say, "Here's the strategy."
Gary Saarenvirta: So, when I work with our retail clients, the retailers say, "This is our business strategy. We want to be number one in produce and meat. So, make sure that your system achieves that goal. We want to always be lowest price in the market. So, we want you to manage competitor price data and make sure that you make recommendations that we're the lowest price in the market." And then give those kinds of strategy rules.
Gary Saarenvirta: So, the human trains the AI with the strategy. And then the AI says, "Okay, I'm not an agnostic to your strategy because you have a brand and a strategy that your company's decided that that's what you want to be." Then the AI will help you make decisions within your strategies.
Gary Saarenvirta: So, it's a relationship between people. People own the strategy and they keep oversight on the system to make sure that if there's any exceptions the humans deal with exceptions. And then now the people can be freed up to work on other things where people are very good at.
Gary Saarenvirta: And, we shouldn't use technology to solve problems that people are good at. Like I'm against customer service robots. That's what people do. I'm against replacing waitresses, and order things, and waiters with automated ordering systems. That's what people do.
Gary Saarenvirta: We don't want to unemploy people. What's going to happen to the world? We can't all sit around and do nothing. There's going to be nobody to make the money to buy the products and services. So, I think a lot of the AI hype has been academics getting a PhD in image detection because that's what's easy to do and thinking there's a business there. And they bring that to the market without thinking of the bigger consequences.
Gary Saarenvirta: I think the ethics are more in the application of AI than in this data bias, which I think is a ... That's only an issue if you use data to build theory. And if you come up with fundamental theories there's no bias in the laws of physics, you know?
Daisy and AI: Letting Humans Do What Humans Are Good At
Kirill Eremenko: Yeah, okay. I see what you mean. And similar I guess to what you were saying before that as a data scientist you should not only look at your system in isolation but the overall effect on the business. Same thing here. AI applications should be valued not just in what kind of impact they have in that specific application but overall what are the ethical considerations?
Kirill Eremenko: And to your point, replacing waiters and waitresses with artificial intelligence might reduce costs but on the other hand ... for me personally ... I prefer to interact with a human. I like that social aspect, social component. And I would probably miss that if all I was doing is walking around every day all day long and talking to robots and being the core super efficient. I like that humans make mistakes sometimes. That's part of being human.
Gary Saarenvirta: Yeah. And I think that's the divide the world in two. What is beyond human capability? Like flying to the moon. We don't try to run to the moon. There's not doubt we're using technology to solve that problem, where we're augmenting our ability and projecting ourselves somewhere where we would otherwise not be able to go.
Gary Saarenvirta: Or let people do what people are good at. That's the dividing line for us. And so, Daisy's mission is to let people achieve their maximum capability by focusing them 100% on people problems. And let's use machine intelligence to tackle the business problems that are beyond a human's capability. That's our mission.
Gary Saarenvirta: And if we do that, we'll change the world because if we augment human capability, it'll create profitability, which will be reinvested back and lower the cost of goods and services. That's the simple theory that I have. And what I see happening today, successful businesses reinvest it back in which serves humanity better.
Kirill Eremenko: Fantastic. I am so fascinated by all these things that you shared. I'm actually real curious. What does a person with such interesting perspectives as yours ... what does a person like that read? What's a book that you can recommend to us that you've read recently or that you really like? I would personally really highly value a recommendation coming from you.
Gary Saarenvirta's Reading Recommendations
Gary Saarenvirta: Yeah, I've been reading a lot of books on business culture. I think businesses like our business succeed based on our employees and our people. Although we're building technology it's still a people world.
Gary Saarenvirta: Some of my favorite business books I've read are Good to Great. Or is it Built to Last? It's a great book about how successful companies and their culture. And recently read a book about Legendary Leadership, which is by [Dan]. I think that's a great book on how to build really powerful cultures and then how to inspire employees to buy into a mission. And I think those are great business books. And then I'm geek so I read physics textbooks and watch ... I'm reading semi-Romanian geometry right now.
Kirill Eremenko: Wow.
Gary Saarenvirta: That's kind of a book for fun. Because I'm trying to lay some more theoretical underpinnings to what we do. Trying to up our game when I someday write a textbook about what we're doing.
Kirill Eremenko: Fantastic. Well, thank you for that list. We'll include all those in the show notes if anybody's interested.
How to Contact Gary and Daisy
Kirill Eremenko: And sadly, this actually brings us to the end of the podcast. I would have loved to continue talking about all these things and, hopefully, maybe you can one day come on the show again. Or maybe we'll meet in person. But I just want to say, had a lot of fun learning from you and understanding this very different and refreshing perspective.
Kirill Eremenko: And before I let you go, please could you share with us where can our listeners find you if they want to maybe connect with you, follow you or, if there's anybody in our audience who would love to consider doing some work with, you and find out a bit more about Daisy Intelligence?
Gary Saarenvirta: Yeah, thanks a lot for having me on your show, Kirill. I really enjoyed it. I love talking about what I do. You could find me on LinkedIn, Gary Saarenvirta. You'll just want to look up the spelling of my name when you post it. Saarenvirta that's one. Our company is daisyintelligence.com. So, you can connect with us there and happily chat.
Gary Saarenvirta: We're always looking for people who believe in our mission, who want to change the world, and customers who want to move to the future. We're there to interest and help them. And anybody who wants to chat about this more, feel free to reach out. It's something we need from a grassroots level to change this discussion that's ongoing. And I'm happy to talk to anybody who wants to participate in that as well.
Kirill Eremenko: Fantastic. Well, there we go. Daisyintelligence.com. Make sure to check it out and connect with Gary on LinkedIn as well. Once again, Gary, thank you so much for coming on the show today. And I can't wait to see how your business grows. It feels to me like you're going to do great things.
Gary Saarenvirta: Thanks a bunch. Appreciate it.
Conclusion
Kirill Eremenko: So, there you have it ladies and gentlemen. Thank you so much for being part of our conversation today with Gary. I hope you enjoyed it as much as I did and got all the valuable insights that Gary was sharing with us.
Kirill Eremenko: If you're interested in working with Daisy Intelligence then make sure to check them out at daisyintelligence.com. Actually, they have 100% ROI guarantee. And I double checked this with Gary and that is indeed the case. So, it's pretty crazy. So especially if you're in the space of retail or insurance, I would be running to give Gary a call and get my hands onto this platform.
Kirill Eremenko: As always you can find the links to all the materials mentioned on this episode including the URL for Gary's company and the URL for his LinkedIn at the show notes, which are located at www.superdatascience.com/291. That's superdatascience.com/2-9-1.
Kirill Eremenko: And finally, if you enjoyed this podcast and if you got valuable insights, valuable perspectives from it then don't just keep it to yourself. Share it with somebody who you know or who you think will also find this valuable. Maybe you know somebody in the space of insurance, or the space of retail, or somebody who's interested in AI and thinks that everything should be just data driven.
Kirill Eremenko: Well, Gary's perspective is that theories are very important too, and challenging your belief systems is always a good valuable exercise. So, I encourage you to send this episode to somebody who you know will be challenged by the insights here.
Kirill Eremenko: And on that note, thank you so much for being here today. I really appreciate you being part of the SuperDataScience community. And I look forward to seeing you back here next time. Until then, happy analyzing.
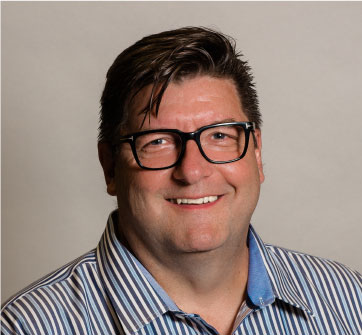
Gary Saarenvirta
Daisy’s Founder and CEO, and a preeminent authority on artificial intelligence and its ability to transform how businesses grow. He is also a member of Daisy’s board.