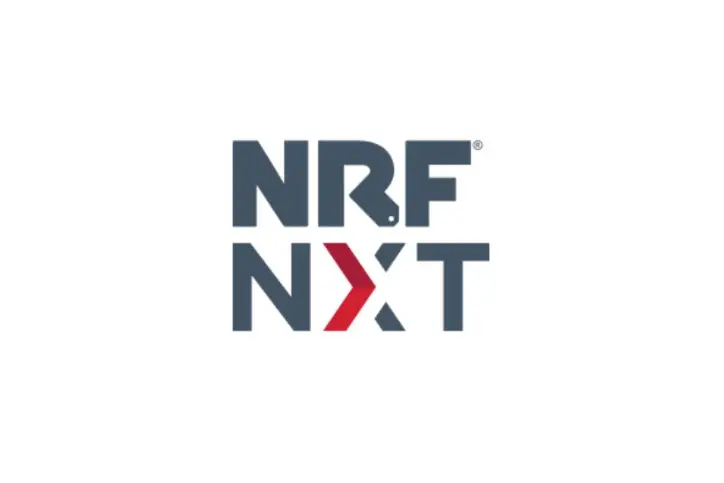
How True AI Helps Retailers Drive ROI from IT Investments
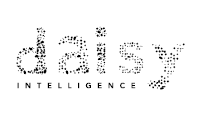
While the technology industry likes to focus on the future and what’s possible, businesses can find it difficult to overcome challenges or problems that happened in the past.
A few weeks ago, we attended NRF Next, the technology-oriented conference produced by the National Retail Federation. There were great sessions on traditional brick and mortar firms embracing digital transformation and case studies of brands beginning online and moving into physical stores as they expanded.
A common thread through many of the conversations, however, was the costly mistakes and poorly-executed IT projects that make retailers cautious about new and innovative technologies.
Many attendees were thinking about companies that were promised major benefits from using business intelligence or predictive analytics applications, only to be told after making significant investments they had a “data readiness gap.” While a grocery or clothing retailer may have lots of data, vendors can claim it wasn’t clean enough and they couldn’t extract meaningful insight.
When these projects fail to deliver, it can be difficult for IT departments to receive the go-ahead to invest in new technologies. As important, it creates assumptions an organization needs clean data before so it can make data-driven decisions.
This might be a good time to confront — and debunk — these assumptions, at least as they pertain to AI based on reinforcement learning.
Traditional data modeling in areas such as predictive analytics requires your data to be labeled, cleansed, and consolidated. This is because predictive analytics models need to be trained.
But reinforcement learning, a branch of artificial intelligence, is not predicated on labeled training data (I.e. you have to have promoted a product or priced it at a certain prince point to build a model). The power of autonomous machine intelligence is, as the term suggests, that it records actions and quickly learns on its own through a trial and error approach simulating to determine the optimal decisions that maximize positive outcomes.
A key part of Daisy’s onboarding process involves ingesting historical TLOG (detailed POS receipts), promotion and product data from which the system can determine key metrics like associated sales, cannibalization, forward buying, seasonality, price elasticity, cross-price elasticity, promotional elasticity etc.
This is different than labelled data because it is not required that the company took an action against a product (I.e. promoted it, priced it at a certain price point etc.). The simulation will use the key product metrics that drives company success without those historical “labels”.
AI based on reinforcement learning allows retailers to see their data differently and more holistically. Daisy’s patent-pending technology helps organizations capitalize on the complex and lucrative relationships between categories and products rather than looking at the business in distinct siloes.
Daisy’s ability to help retailers drive ROI is grounded in taking a different and better approach to fundamentals areas like selection the optimal promotional product mix, promotional/regular pricing mix and demand forecasting/inventory allocation..
The bottom line is that AI based on reinforcement learning unleashes new approaches to decision making throughout an organization. This is an important initial step for retailers so they can move forward with confidence about strategic and tactical decisions that drive future growth.
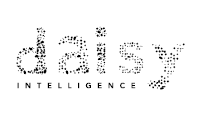