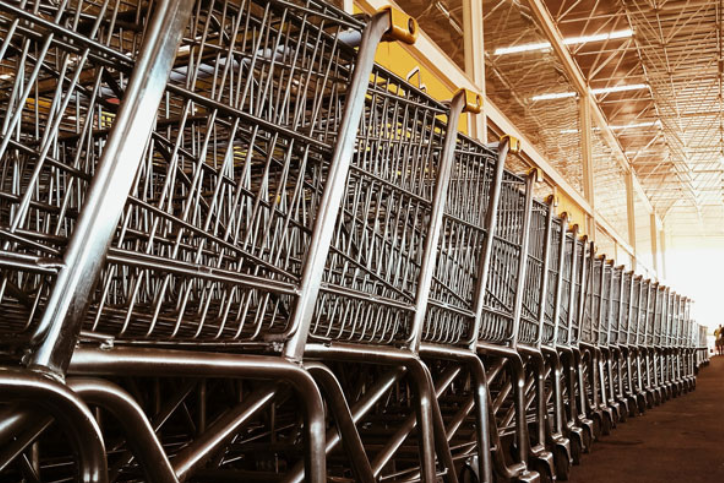
COVID-19: The End of Predictive Analytics in Retail
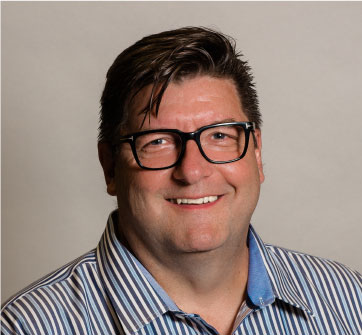
A few years ago, I contributed to an article in the Q2 2017 issue of Platt Retail Institute’s Journal of Retail Analytics titled “Comparison of Traditional Predictive Analytics Tools Versus Artificial Intelligence-Based Solutions.” In it, I wrote that, “In this day and age of radical disruption and revolutionary changes in retail, predictive analytics models are not really up to the task of looking at a retailer’s data holistically and supporting profitable decision making.”
Three years later, COVID-19 has now for all intents and purposes rendered traditional predictive analytics obsolete in retail.
Predictive analytics is the typical approach that most retailers use and considers past data points only to forecast and determine which products to promote and at what prices. This approach has historically added value, but it relies on customers behaving mostly in similar ways week after week, year after year. Predictive analytics requires historical trends to be able to predict what will happen in the future. In fact, forecasting weekly SKU sales typically requires at least a few years of data.
COVID-19 has broken customer shopping habits and historical trends. Retailers will not be able to rely on historical trends to understand the current landscape and adapt to ongoing change. Predictive modeling, by extension, will become less and less relevant. However, successful retailers will still need to invest in the ability to scenario plan with agility in order to run their businesses profitably.
Daisy’s approach is different. Our technology is based on what is known as “optimal control” or “reinforcement learning,” where the governing mathematics are independent of historical trends and data and instead act like the laws of physics that govern our universe.
The optimal control approach still uses retail data but only to calculate changes in product features such as halo sales and cannibalization, and how the items will behave under the retail laws of physics or Daisy’s Theory of RetailTM. This allows us to rapidly build scenarios and forecasts built on millions of optimizations that are in turn based on changes in product features. Understanding the dynamics of items requires as little as a few weeks of data—not years of historical trends. Retailers cannot wait years for predictive analytics to build up that knowledge (or as we like to put it to “learn at only the pace of time”).
Over the past 50 years, retail analytics has advanced from business intelligence to predictive analytics, and now to the emergence of autonomous artificial intelligence. These turbulent times only reinforce the importance of being able to plan for the future based on the dynamics of retail rather than what has happened in the past.
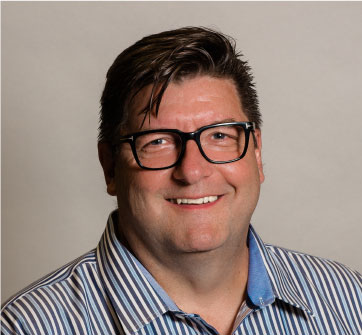
Gary Saarenvirta
Daisy’s Founder and CEO, and a preeminent authority on artificial intelligence and its ability to transform how businesses grow. He is also a member of Daisy’s board.