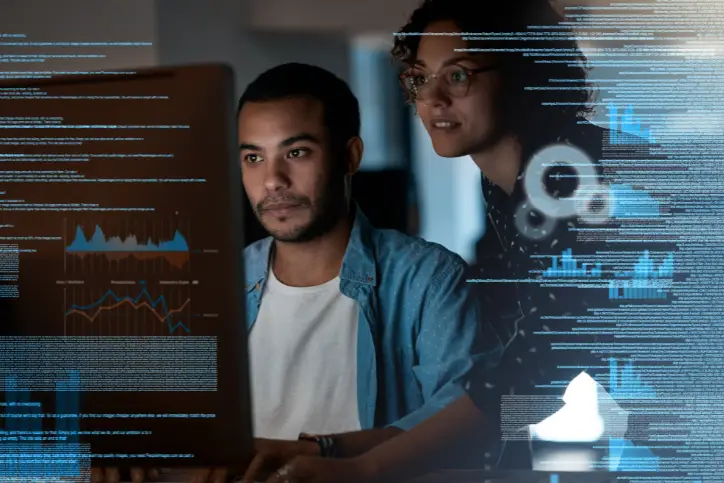
Explainable Decisions-as-a-Service for Retail and Insurance
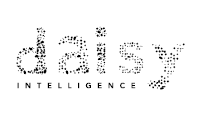
It is no secret that artificial intelligence (AI) is transforming numerous industries, providing organizations with a competitive edge and enabling them to outperform their competition.
In particular, AI that delivers explainable decisions is rapidly driving companies to success. Delivering explainable decisions builds trust in AI-powered recommendations and ensures the transition to autonomous operation is successful - allowing organizations to fully reap the benefits AI offers.
This blog post will provide a comprehensive introduction to AI that delivers explainable decisions, defining the concept, how it compares to traditional tools, the benefits it offers, and how the AI is being applied in the retail and insurance spaces.
What Are Explainable Decisions?
Explainable decisions are AI-powered decisions that are delivered with context - allowing the personnel using the AI system to fully understand the logic behind the recommendations being provided. Explainable decisions mean that the AI system is not a black box – there is visibility into the way the system operates and the basis for its decisions.
Explainable decisions leveraged in retail and insurance are used to develop trust in AI-powered decisions as organizations transition from using traditional technologies and methods to automating core processes with AI systems.
Trust is the basis for success in any AI implementation effort as AI is not a project, it is a significant transformation initiative and will change the way an organization operates. It is not in human nature to accept such significant change with no understanding in the technology that is taking over day-to-day responsibilities. As such, explainable decisions are a necessary component to ensuring the success of AI implementation efforts.
Explainable AI VS. Traditional Tools
The main point of differentiation between traditional advanced analytics systems and explainable AI systems is the level of transparency and visibility. As aforementioned, AI that delivers explainable decisions provides full visibility into the internal logic of the system – providing users with the rationale behind all outputs and decisions.
However, the same cannot be said for traditional analytics tools. Many of these traditional systems are black boxes – only permitting visibility to the inputs and the outputs of the system but providing no clear visibility into the processes in between. Even when end-users are allowed a look into the inner workings of the system, they are typically unable to gain any deep understanding due their lack of expertise with the technology.
The Benefits of Explainable Decisions
In addition to the emphasis placed on providing a clear rationale for all recommendations, AI that delivers explainable decisions comes with several other benefits.
Most notably, these systems require no code, infrastructure, or data scientist personnel and these systems can integrate into existing back-end processes, such as robotic process automation (RPA) systems in the case of insurance. These systems will also automate and continually process the most up-to-date data as they are extremely dynamic - operating and learning faster than the pace of time.
Furthermore, building trust in AI technology with explainable decisions has the power to elevate the role of people in the workplace. When trust is built and the AI system is allowed to take on the mundane tasks it is far better suited to do, workplace personnel see significant time savings. This time will ultimately be reinvested and will be used take on the high value tasks only they do best – this includes business strategy and meeting customers’ needs.
Explainable Decisions in Retail
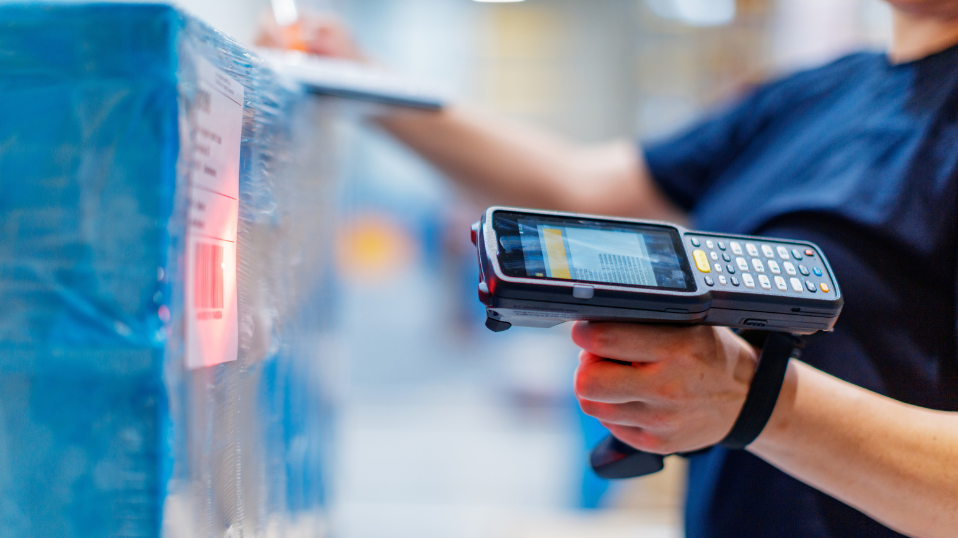
In retail, an explainable decision may look like a rationale for why a certain price point was recommended. For example, suppose the AI system recommends that ground beef is promoted at an unexpectedly low price. With no explanation, this recommendation may appear to be a mistake – making retailers wary to accept and action the recommendation.
However, when the decision is explained (the low price point will increase customer traffic and subsequently drive the sales of products related to ground beef, such as pasta and tomato sauce), it becomes clear that the decision was well justified – the retailer will recoup the margin loss on the ground beef with the associated sales of the other products. With each explanation, retailers will reach a greater understanding and trust that the thousands of decisions across their store are being made correctly.
A typical retail store houses thousands of products – resulting in millions of possible item and price combinations. Making decisions of this magnitude for all products across an entire store is a task that is a measure beyond human ability. Building trust in AI means these decision-making tasks can be turned over to the system, alleviating the strain on the retailers making these impossible decisions.
Explainable Decisions in Insurance
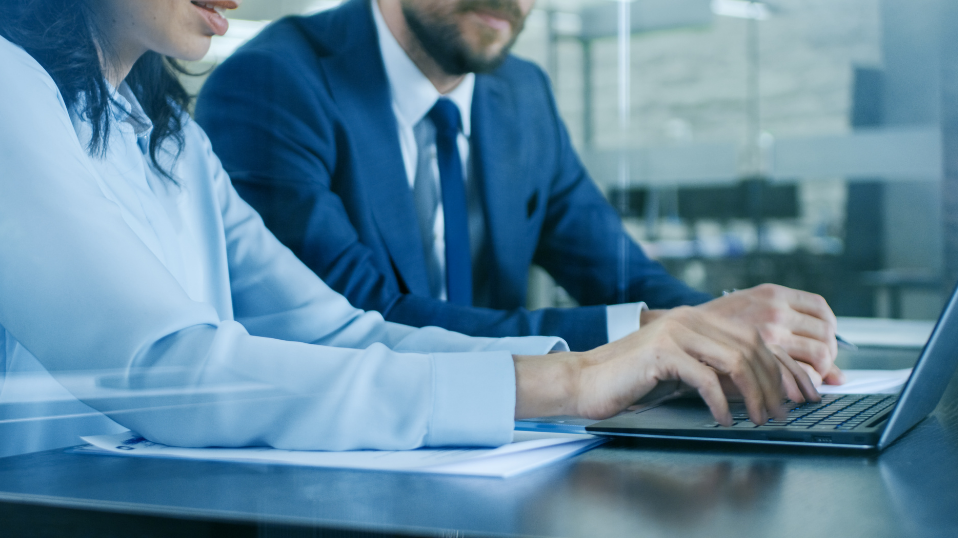
In insurance, an explainable decision may look like a rationale for why a claim was denied due to fraud. Suppose a claim for prescription medication was deemed fraudulent by the AI system. With no further explanation, it may seem incorrect that this claim was flagged as fraud – there is nothing inherently suspicious about a low value claim for medication. What’s more, insurers that are not leveraging AI systems might even process this claim, as it is not cost effective to investigate further.
However, when the logic behind the AI’s decision is revealed, it becomes clear that it was well justified. In this case, the decision was founded on the fact that the prescribing doctor is writing prescriptions too frequently in comparison to multiple sets of peers (such as similar specialty doctors, doctors with practices in the same area, or doctors prescribing to the same age of patients at the same time of year). Behaviour that is anomalous compared to multiple peer groups makes the case for fraud and abuse clear. Once again, with each explanation, insurers begin to feel confident and trust that the decisions across their organization are being effectively handled by the AI.
AI has rapidly reached every industry and is becoming the key strategy for success among retailers and insurers today. As such, organizations looking to compete and lead their industry are quickly investing in AI technology and implementing it internally to automate core processes.
Leveraging AI that delivers explainable decisions is a sure-fire way to ensure AI implementation efforts are successful. Explainable decisions work to establish trust in AI-powered recommendations – making the technology far easier to accept. AI that delivers explainable decisions is also incredibly dynamic, outperforming traditional tools and elevating the role of people in the workplace. The end result is time savings of 40% and a ROI of >10X.
Ultimately, implementing AI is a significant transformation effort. As such, it is important end-users understand and believe in the technology they are using. The transparency explainable decisions provide will be the driving force behind long term success – ensuring retailers and insurers can continue to compete and provide customer value.
Learn more about the technology that is enabling Daisy to provide value in the retail and insurance spaces. Get in touch.
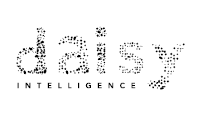