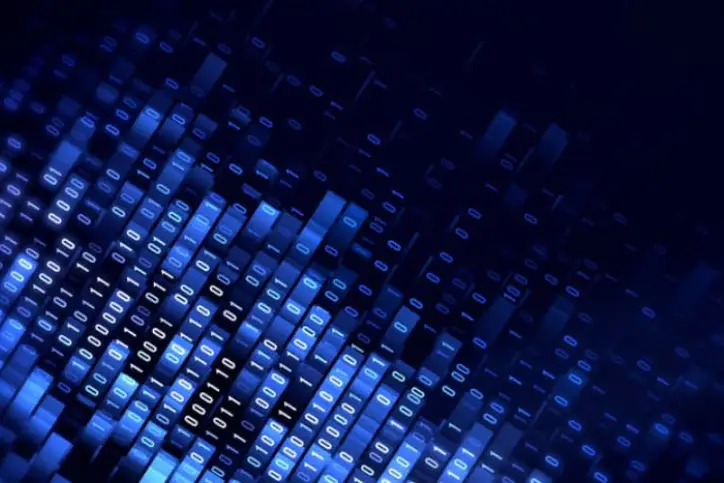
Reinforcement Learning, the Right Technology in Retail
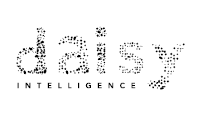
Many of us who have been in our careers for a while have probably never taken a formal course in AI. In fact, even today, less than 50% of high schools in the US even offer a course on the subject (though that is changing quickly). This means most of us have a limited understanding of what AI is, how it might be applied in our business today, and how it will affect our roles in the future. This is a perilous position given the considerable impact it’s having today, and the tremendous growth predicted over the next 10 years.
The Use of AI in the Retail Space
At the end of this decade, the global financial impact of AI is expected to reach 15-20 trillion dollars and it will fundamentally impact nearly every aspect of business. For senior retail executives, it is vital they lead their organizations through this change rather than follow from a place of unfamiliarity. To do so, their organization, and they themselves, will have to acquire a comprehensive understanding of AI and the opportunity it offers their company. The urgency in bricks and mortar (B&M) retail is especially great as this sector has historically been a laggard when it comes to technology. That said, when combined with ecommerce, it is now poised to overtake the banking industry as the top spender on AI. B&M retailers have specifically accelerated their spend, adopting AI technology for a wide range of tasks including inventory management, in store experience, and merchant planning of promotions and pricing. According to a 2021 survey by Meticulous Research, the projected growth of AI in retail will be at a pace of 35% CAGR over the next five years.
There are a several branches under the general umbrella term of AI. However, the application that is rapidly becoming the most important by far for merchants is machine learning. Machine learning is the use and development of computer systems that are able to learn and adapt. This is done by developing and using models, which fall in to three categories: supervised, unsupervised, and reinforcement learning.
In merchandise planning, reinforcement learning (RL) is emerging as the dominant technology. The key reason for it's prominence is its ability to autonomously handle massive amounts of data, countless variables, and incredible levels of complexity without requiring much human labour. The technology keeps humans in the loop, but only to guide the technology to follow the strategies of the company and to evolve the technology itself further.
What is Reinforcement Learning?
We are intuitively aware of how positive and negative reinforcement work in our everyday lives. For example, a parent may give their child praise after they studied or finished a chore in the hope that this would become regular behaviour. That is a simple everyday example of positive reinforcement. On the other hand, an example of everyday negative reinforcement would be being reprimanded for arriving at work late. Leaving earlier would be an action learned through negative reinforcement.
Moving to the world of retail, let’s take an example of a merchant who is brand new to her role. Her strategy of placing hamburger patties on promotion is rewarded with increased customer traffic to the stores, hamburger patties flying off the cooler shelf, and boosted sales of complementary items such as buns, ketchup, and cheese. Clearly, the novice merchant will take note and repeat this promotion after having received positive reinforcement from the results. In fact, one of the key attributes of a successful merchant is their deep knowledge and experience of the hundreds of promotions that work and the ones that don’t, which is learned through positive or negative experience throughout their career.
In principal, reinforcement learning in AI works the same way - but any comparison on performance quickly becomes obsolete. Unlike merchants and their analytical team learning in real time, the AI is continually learning autonomously at lightning speed from billions of positive and negative reinforcements in a virtual retail simulation of the entire business or system. This approach explores not just the relatively small number of promotion scenarios that a merchant and their team is able to accomplish, it considers all potential product combinations, potential price points, and channels to provide fully optimized and holistic recommendations.
The Future of Reinforcement Learning
Rapid adoption of reinforcement learning is beginning to provide great successes. The board game Go provides a glimpse into the future of this technology in retail. Go is a board game invented in China more than 2,500 years ago and is considered the most complex game ever devised by humans. DeepMind (a subsidiary of Google) has been evolving an AI program called AlphaGo that leverages reinforcement learning. Since the early 2010s, DeepMind has hit the headlines for their incredible progress. In 2016, one of their first versions was able to beat the world champion Go player 4 out of 5 times. The next iteration, called AlphaGo Master, defeated not only the reigning Go champion, but also 60 top professional online players without losing a single game. Incredibly, the latest version of the program, AlphaGo Zero, played the previous version AlphaGo Master and defeated it 100-0.
This very same approach is now being harnessed by retail leaders who understand the power of AI technology. For senior retail executives, it is vital they understand the technology and lead their organizations through the transformational change they must undergo. They will not want leave their organization unprepared to compete against the retail version of AlphaGo.
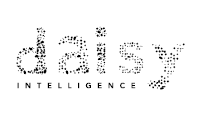