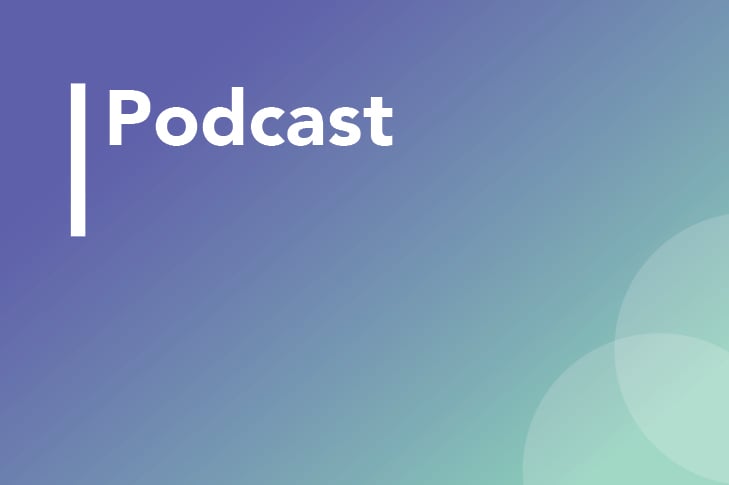
Gary Saarenvirta on the AIP Podcast
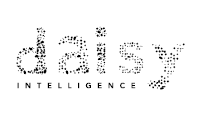
Watch The AIP Podcast
Daisy Founder & CEO Gary Saarenvirta joins Anne Cheng, CEO & Founder of Supercharge Lab, on the AI Partnerships (AIP) podcast, discussing Daisy's AI-powered platform for retail merchandise planning.
Original source: AIP Podcast Ep.18 from AI Partnerships Corp. on YouTube.
AIP Podcast Transcription
Anne Cheng: Hey, good morning, good afternoon, and good evening. Welcome back to yet another episode of the AIP podcast. As usual, it's your host Anne Cheng of Supercharge Lab. On behalf of AIP, the AI Partnerships Corporation, I want to give a really really warm welcome to the new and returning listeners. Thank you everybody for tuning in today. Guess what? We have the amazing Gary Saarenvirta with us. He is the CEO and Founder of Daisy Intelligence. Daisy Intelligence is a disruptive AI-based SaaS company that delivers business optimization services to unlock insights from transactional data to help retail and insurance customers achieve better bottom-line results. Daisy Intelligence specializes in improving promotional effectiveness, product planning, product selection, customer loyalty, core merchandising operations, and even fraud detection. So, let's jump right into it. A huge warm welcome to you, Gary, it's our pleasure to have you here today.
Gary Saarenvirta: Great. Glad to be on the podcast today.
How Was Daisy Started?
Anne Cheng: Absolutely. Well Gary, let's talk a little bit about your background. How did you get started with Daisy Intelligence?
Gary Saarenvirta: Well, I'm an aerospace engineer by education. I have a graduate degree in that from the University of Toronto and when I graduated there's not much of an aerospace industry in Canada. So, I didn't want to move to go pursue that career, so I found this accidental opportunity working for big corporations to bring more math and science to decision-making. You know, coming from the engineering world, the aerospace world, I was shocked at how little math and science was used in big companies. So, I’ve created this accidental career for myself, and I've been doing this now for close to 30 years.
How Does Daisy Help Businesses Make Better Decisions?
Anne Cheng: Wow, that's amazing. So, how does Daisy help businesses to make better decisions? To put it into context, a lot of senior leaders today will boil it down to experience or even the understanding of human connectivity that really drives the best outcomes. Do you really disagree?
Gary Saarenvirta: Yeah, I think its data driven. I think there's certain business decisions that are really beyond human ability. So, for example in retail, if you're a retailer with a hundred thousand products, which would be a typical hyper market retailer, there's a hundred thousand squared patterns or interactions between products because customers don't buy items, they buy solutions, and solutions contain multiple products. So, for example, a grocery example that I always use is a customer who buys ground beef to make a pasta dinner will buy pasta, tomato sauce, bread, cheese, wine, salad fixings. The use case for ground beef requires other products to be purchased. And so, those Halo relationships become almost infinite. So, you have billions of those combinations and product relationships, and to optimize that, to choose the optimal combination of products to promote, is really beyond human ability. That's where the technology comes in, helping decide what should you promote and what do you not promote. Because if you promote a turkey and the consumer buys all the Thanksgiving dinner fixings, you don't need to promote all the fixings, the consumer will buy those. It's unlikely that they will come and just cherry-pick a turkey. So, it's what to promote and what not to promote are the key decisions, and that's what the technology can do. Because the combinations are almost infinite, it requires technology to help the business.
How Autonomous is Daisy's Solution?
Anne Cheng: Certainly. You mentioned during our pre-call conference that you talked a little bit about AI-powered decisions for merchandise planning and risk management. You also mentioned that your Decisions-as-a-Service solution works across all industries. So, tell us a little bit more about how autonomous your solution is. Especially in this very extraordinarily tedious function of data labeling and data ingestion from disparate sources, which can even include things like pieces of paper, CD-ROMs in a bunch of drawers, how do you deal with this?
Gary Saarenvirta: I mean, we use the electronic data. If you're optimizing transactions and trying to improve transaction processing, it's information about transactions that's most useful. And so, we don't use – you know, when most people say AI, it's really predictive modeling statistical analysis, human lead, a human being hand crafting models using sophisticated algorithms. Our technology is more based on reinforcement learning, or more what the engineering community has been doing for 50 years in aerospace. So, we deliver the decision. There's no human in the loop. To be AI, it should really be autonomous with no human in the loop. If there's a human being building models, it's really human-led analysis. So, the idea of artificial intelligence is that it's completely autonomous, and that we don't have machine learning data scientists who are handcrafting models. We've invented a mathematical theory of business that the system can simulate the future. If I was to give you a couple of analogies of what our tech is like, a modern fighter jet is not actually flown by the pilot. He or she puts the objective into the plane's computer by pulling on the joystick, rudder, pedals, throttle, and then the computer translates that into flight surface adjustments. So, they adjust the flight surfaces a thousand times a second, which is beyond human ability. So, the human sets the objective, and the computer takes care of the gory details. A second analogy would be that of a race car. If you were to build an autonomous race car, you wouldn't teach it to drive by building a physical car with an empty brain, putting it on the racetrack, and say learn to drive. You would crash a million cars. So, how do you train a race car's brain? You build a simulation of the world. And so, in the case of a race car, you have the laws of physics. There's no historical data, that's labeled data, that you'd say this is a good millisecond, bad millisecond, in building a predictive model on how to drive a car. That's not how it's done. You would simulate the world. The historical data would configure the simulation, so you have the laws of ground, you have the gravity, friction of the rubber on the road, air density, and then the computer would say what's the optimal sequence of steering, brake, and gas pedal inputs that generate the fastest lap time. And so, that's a second analogy. So, for us, a year of business is like a lap around a racetrack and it's the optimal sequence of decisions that you make to control profitability. So, in retail, it's the weekly sequence of what do I promote, what do I not promote, what prices do I charge, how much inventory do I allocate. In insurance, it's the weekly sequence of underwriting decisions. So, what premiums do I charge for my policies, should I pay this claim or not, adjudication, is this claim fraudulent or not. So, it's a sequence of decisions that our system does autonomously with no human in the loop, and that's what we call Explainable Decisions-as-a-Service. And so, you know that making those decisions is what impacts company profitability and that's, for us, the definition of AI. This is what's known as reinforcement learning or optimal control. And so, our technology is very similar to autonomous cars or drones in that there's no human in the loop and it's a system that's making all the decisions. Then the key thing for our clients is that you have to explain why the decisions are being made. Why am I promoting a particular product, or why is this claim deemed to be fraudulent. So, it has to come with an explanation. And so, our system will provide an explanation for the human being that does want to have a look and feel that they trust the system, because building trust is the most important thing when human beings are involved. They will not just turn over corporate decision-making to a technology platform without understanding what it does. So, explainability is critical. And that's a big part of what our system also does. It says this is why we promoted the product, this is why this was the price, this is why we should auto-pay that claim, this is why you shouldn't pay that claim. And then once the human beings build the trust, then they can release some of this automation and let it happen on its own.
Daisy's Risk Sharing Business Model
Anne Cheng: Sure. That sounds amazing. And you also mentioned something very interesting during our pre-call conference. You said you participated in risk sharing through your business model. Have you had a lot of upside benefit in this risk sharing model? And have you ever shared in the downside of risk as well?
Gary Saarenvirta: I mean, if you make better decisions, you will have better outcomes. So, in retail, if I promote a better set of products, and I have a better set of prices, and I have a more accurate inventory available in every store, there will be value created. So, every single client who we've worked with, who has acted on the output of our system, we have created incremental profitability. And so, we believe in our technology so much that we're willing to do risk sharing. Many of our clients take us up on that offer because it aligns our interests as a technology company. We're not just selling our technology and then you figure it out, we're aligned in the success of our clients. And so, we have never had downside risk, it's always been positive because it's simple. Better information, better decisions, equals better outcomes. We see that in every single industry. So, many clients want to share the risk because of that alignment of interest. Some clients just say, no, we're happy to pay your fees, we want to get all the benefits, and we're okay with that model too. But it always works because good information equals better outcomes, that's really a fundamental truth.
How Does Daisy Help Offline Retailers with Increased Pressure from Big E-commerce Players?
Anne Cheng: That's great. You talked about working in retail. So, how do you help offline retailers who deal with many human aspects to drive outcomes in a world where e-commerce players, like Shopify and Amazon, are now becoming more competitive than ever, especially post-pandemic?
Gary Saarenvirta: Yeah. We work with bricks and mortar retailers and with e-commerce or omni channel retailers because the same truths hold. Consumers buy solutions. Whether they're buying the solution online, its the same product use case. If a customer is buying a box of cheerios in the store or online, they will buy the same products with it because to eat cheerios most people buy milk. So, they'll buy milk and cheerios together. Maybe they'll buy some fruit to put in. So, the customer usage pattern is independent of how they purchase the product. So, those same opportunities exist to maximize on those use cases regardless of the channel. So, I think by helping bricks and mortar retailers be more profitable, that helps them compete against the online retailers or in the omnichannel world. Now that e-commerce has grown, we know that e-commerce for bricks and mortar companies is a less profitable channel. So, anything to create increased profitability will help them continue to grow and thrive. And so, it's important. Making smart decisions creates better profits. Smart companies don't bank the profits, they reinvest in innovation, and they reinvest in price. So, our ultimate goal is to see consumers get the lowest prices possible and allow their stores that they shop at, whether online or bricks and mortar, to be more competitive. Even in insurance it's all about being price competitive, it’s one of the key drivers of success for most companies. So, this type of technology allows companies to be efficient and offer the best prices to the market.
How to Get in Touch with Daisy
Anne Cheng: Absolutely. Well, that's just about winding up for the rest of the show. So, maybe we can just share with our listeners how we can get to contact you, Gary.
Gary Saarenvirta: Yeah. You can come to our website at www.daisyintelligence.com. You can also look me up on LinkedIn. I'm more than happy to connect and respond to you there. My LinkedIn name is Gary Saarenvirta, I’m one of the only Saarenvirtas in the world, so you should have no problem finding me, and you can see my name on our website as well. So, feel free to reach out, happy to connect. I love talking about technology, and if we can help your business, that would be even better. But feel free to reach out.
Anne Cheng: Absolutely. Well, Gary, thanks so much. What a thrill to have spent some time with you today. Once again, thank you for your time, Gary. And to all our listeners, thank you so much for tuning in. This is Anne from Supercharge Lab. Once again, on behalf of the AIP podcast, signing off.
Gary Saarenvirta: Thank you, it's great to be part of the show today.
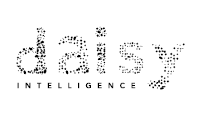