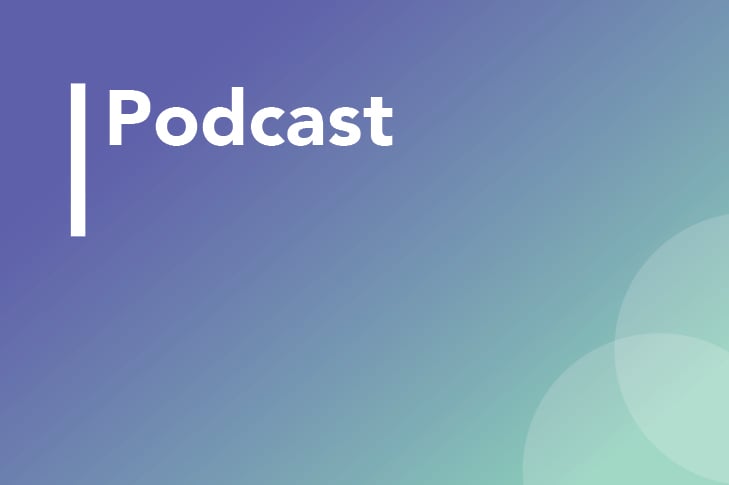
Gary Saarenvirta on the Retail Tomorrow Podcast
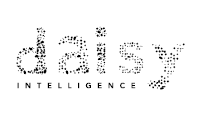
Listen to the Retail Tomorrow Podcast
Daisy Founder & CEO Gary Saarenvirta joins the Retail Tomorrow Podcast with co-hosts Sterling Hawkins and Kevin Coupe to strip artificial intelligence down to its most basic parts.
Original Source: Retail Tomorrow Website.
Retail Tomorrow Podcast Transcription
Kevin: October 27th, 2020. How can AI and machine learning help retailers be more efficient and effective? What's the best way to compete using AI? And, as it becomes more mainstream, will it even be possible to compete against AI? Should we think of AI in terms of Star Wars C3PO, or like the Terminator? Those are just some of the questions as we look into the possibilities and potential of Retail Tomorrow.
Kevin: Hi. I'm Kevin Coupe, content guide for morningnewsbeat.com.
Sterling: And I'm Sterling Hawkins, co-CEO and co-founder of CART, The Center for Advancing Retail and Technology.
Why Was Daisy named Daisy?
Kevin: Our guest today is Gary Saarenvirta, the founder and CEO of Daisy intelligence and a preeminent authority on artificial intelligence and its ability to transform how businesses grow. Now, as I was doing my research and trying to formulate some intelligent questions, it occurred to me that in 2001: A Space Odyssey, when astronaut David Bowman is disconnecting the Hal 9000 supercomputer, which had artificial intelligence, he had Hal sing a song that it had learned during programming, Daisy. You know, Daisy, Daisy, right? That's the song. Now, I thought this was not just very cool, but it would give me a chance to, kind of, throw in a quick plug for a book I co-wrote, The Big Picture Essential Business Lessons from the Movies. But then I did a little more searching, because the Google machine, you know, it's really wonderful. And I discovered that, in fact, in 1961, at Bell Labs, an IBM 704 mainframe computer was actually taught how to sing Daisy, in what was the earliest demonstration of computer speech synthesis. I had trouble saying that word. So, in other words, 2001 was doing an homage to a real event, which is a long way, a really long way to get to my first question. Gary, is that why you named the company Daisy?
Gary: Absolutely. Those are two of the reasons behind the Daisy, and then the third one being, you know, the number of petals in a daisy flower is a Fibonacci number. So, this is what our ad agency came up with. I asked him to watch an episode of Nova called the Great Math Mystery. I said, I wanted a name like apple, watch this episode. And they came back with those two stories. And the fact that daisy flowers, the number of petals is a Fibonacci number. And that, like, hit me like a ton of bricks, and the name is Daisy intelligence. There you go.
Kevin: How great is that? I was deathly afraid that you were gonna say, oh no, it's not that, that was, that was my daughter's name.
What is AI Really?
Sterling: I love it. I have to watch this Nova Great Math Mystery. I think I've got my weekend plan, or at least part of it. But Gary, let's start somewhere that I think is probably pretty easy, but something that I think we're probably all screwing up. You know, AI is everywhere. What is it actually?
Gary: Yeah. It's, I mean, there's a lot of definitions. I'll tell you our definition, right? I'd say for the most part AI has been statistics rebranded. So, predictive modeling, you know, and also, I’ll contrast what, what our definition differences between predictive modeling. So, predictive models, you need labels, which means you need to have history, you know, you need to have experienced prior history. And then you model that prior history and try to predict labels, like you look at all the customers who bought a product, and then you can say, do these new customers have the same attributes as those other customers that bought Coke? And then say, they're likely to buy Coke, and I can now send them a coupon. So, it's modeling previous history. And I'd say, for the most part, when you hear labeled data, you need lots of labeled examples and this big sea hunt for big data, then you're really just doing statistics. You know, linear regression was invented in 1805. Deep Learning is just very sophisticated linear regression. If it was the panacea, it would have run its course. With predictive modeling, you can only do what you've ever done in the past. Because you have to have those labels. And therefore, you can only learn at the pace of time, which is, you have to do something, measure it, then build a model. So really, you're learning only at the pace of time because you have to go test something new in the market. And so, contrast with that, kind of, what we do is what the aerospace industry does, which is called reinforcement learning or optimal control. You know, you don't fly a fighter jet with, with a predictive model. A fighter jet is actually not even flown by the pilot. The pilot puts the objectives in, he or she, you know, pulls the joystick, rudder pedals, the throttle to tell the computer where, where he or she wants to go. And then the computer adjusts the flight surfaces 1000 times a second. And so, you know, and a fighter jet would crash if, if the computer wasn't flying it. We call that kind of autonomous machine intelligence with human oversight. And, you know, that's, kind of, you know, one analogy that we use to describe AI.
Kevin: Suddenly, that changes Top Gun totally. Now you’ve just destroyed that entire image of Tom, Tom Cruise flying.
Sterling: So many movies in this episode.
Kevin: I'm sorry.
Gary: Yeah. Well, the computer crashed, Tom would have ejected because the, because the plane would have gone into a flat spin. Because it's unflyable by a human being, right? That's, that's the point, is that AI is doing something that's beyond human ability. And so, the other analogy we use to describe the tech would be like an autonomous race car. So, in an autonomous race car, again, you don't drive with a predictive model you, there's a new track, and there's no statistical model of every millisecond is either good or bad to create a label. You, you would, you wouldn't put a brand-new car on the road with a with a, with a brain that hasn't learned to drive because you'd crash a million cars. So, what do you do? You train the car in a simulator on how to drive. And that means you have to have a simulation of the world. And in that case of a car, it's the laws of physics. And you do use historical data, but you use that to configure the simulation. So, you would want to know what the air density is, what's the friction of the rubber on the road? What's gravity? And you plug that into your simulation. And then the computer tries to figure out what's the optimal sequence of steering, brake, and gas pedal that gets me the fastest lap around the racetrack. And, you know, that means, and you can run that simulation faster than the pace of time, you can do 100 million hours of driving in one hour. So, you're learning faster than the pace of time. You have no history, so you can do things that human beings have never done before. And the system is autonomous, it's completely in control, it tries to figure out the optimal sequence. It's not, there's no, there's no extra step. In predictive modeling, there's an extra step that says, if I have this model result, what should I do? An autonomous system is self, self-encapsulating. And so, I'd say that that's for us what AI is, the ability to do what we've never done before, learn faster than the pace of time. In fact, if you have enough computing power, doing 1000 years of retail in one hour, and recommending what to do, like is running the system. And so, for us a year of retail is like a lap around a racetrack. And when, instead, to drive a retailer, it's the optimal sequence of what products to promote, what products not to promote, what prices to charge, how much inventory to allocate. Those weekly decisions is how you drive a retailer to profitability. And so, that's our definition of our tech. And our tech has the merchant and the category managers, like the pilot, they set the objectives. You know, the computer is not going to tell a retailer, hey, you should be EDLP or high low. Or, hey, you should be, your brand proposition should be number one in fresh. Or, you know, the computer's not gonna do that. That's what the role of the human, the humans the pilot, they're the boss. And the AI takes care of the gory, gory details that are beyond human ability, which is figuring out, of your hundred thousand products, what should I promote, not promote? Out of my 400 stores and hundred thousand products, what prices should I charge? How much of every product should I have on every shelf? You know, those kind of things, that's beyond human ability to do that effectively, right?
Sterling: That's always blown me away, is how much faster and better AI can be, at least real AI. And what was the number? You told me once, Gary, or I saw it somewhere. It was like, you do in a day what would take a whole team of humans like a hundred thousand years, something like that.
Gary: One of the examples is, you know, we have, one of our clients said tell us everything you know about one, one SKU, right? And so, that was a, was a PowerPoint presentation that was 100 pages long, right? That's what the AI knew about the SKU. And then we said, well, you got 100,000 of those. So, am I going to make you a 10-million-page presentation to understand what you're, what you do? So, how long would it take all your merchants to read a 10, 10-million-page presentation? And by the way, it's a new one every week because everything changes like every day. So, yeah, it's way beyond human ability. Figure out those gory details, but the human can figure out, you know, what's our strategy? What's our, what are new innovative products? How should I service my customers? I can negotiate with my vendors. Those are the things that, that, you know, that people are great at that computers are not good at. But computers are good at crunching, crunching math and numbers. You know, that's, that's the difference. And we see the, dividing the world into let people do what people are good at, let computers do what computers are good at.
Kevin: It's also, it was interesting, there was a story of, within the last week or two, about how Kroger has created this new app. Chef Bot, I think they call it. And basically, it uses AI. If you have the app on your phone, you can go to your kitchen, take a picture of three, three ingredients from your refrigerator, your freezer, your cupboard, send it via the app back to them, and they use AI to give you a recipe that uses those three ingredients. And that sounds beyond just crunching math to me.
Gary: To me that example is not AI, that's like a search, you know. What recipes have these three items in it? You know, that's not, I'm not sure that, you know, that's an example of where everything is not AI just because it's tech. You know, I saw an article, or somebody wrote, you know, we see these, you know, tablets in restaurants, man, that’s a really cool application of AI doing ordering. No, that's just technology automation, right? So, you know, it's like, not everything is AI just because it's technology, I think it's those distinguishing features. Is it, is it autonomous? Is it doing something people can't do? Like, you can give me three, three ingredients and I'll give you a recipe. You may not like it, but.
Kevin: That goes back to Sterling's first question, which is, to phrase it another way, are people calling things AI that really are not AI? And it sounds like the answer to that question is a big yes.
Gary: Absolutely. Because there's really not a clear definition. It's like, say, like, saying AI is like saying language. Okay, well, what is that? What is language? Well, yeah, there's like 700 languages, and there's like 14 dialects of each language. And, you know, the, you have to get more specific. But I get, the definitions get so technical. Like even my description, I, you know, of what I think AI is, is that, is that tractable to the average layperson? I don't know. I'm like so technical, I sometimes don't know if I'm bridging that gap or not, right? So, it gets very technical. So, it's easier just to say, hey it's AI. I actually hate that term. I think that's a terrible, terrible term. It's all computer programming. It's math and science. You know, it's, it's not space age, as people think it is.
Kevin: Because I would have thought that AI was, would go beyond math and would actually, ok, we're getting maybe into the realm of science fiction, but AI actually allows a, allows a computer to think in a different sort of way.
Gary: No, it's really, I mean, it, that's another misnomer. It's, it's all math and science, it's all programming. So, what, what, what, what people think is the thinking, it's like randomness, right? It's, so like an example of when you were a child, and you had those blocks where you're trying to put a square block into a round hole. You had a little panel of holes, and you had a pile of blocks. And so, little, you watch kids, they randomly try to fit the block in the hole, they eventually get it right, then they start all over again, they still do it randomly the second time, because they don't remember. And so, over time, they do it more, and more, and more. And eventually they learn. And so, that's called reinforcement learning, you reinforce your experiences interacting with the environment. And there's an element of randomness to that. And so, you know, that's, that's the, kind of, motivator for this, kind of, type of AI that I talked about. But it's, it's basically just putting a random number generator in a piece of software code that generates a random result. While probability and was invented in I think, 1654, we know how probability behaves. So, putting a random number generator in a piece of code, that doesn't make the computer think. And then through brute force, the fact that I can do, you know, I can play 100 million games of GO, is it surprising that you would beat a human, a human being who can only play 10,000 games in their life? Like, you know, it's brute force computing, combined with doing things, and just doing more than a human being can ever do. So, to call that thinking, I don't know. Is that thinking? You know, maybe a philosopher would say yes, of course, that's thought. And I don't know. For me, it's brute force computation. And so, it, kind of, it, kind of, in my mind, it's not as exciting some of these advances, it's more about the computing power has increased. Its Moore's law that is driving these advances, not any sophistication in algorithms or software. But that's my opinion, right?
How Does Daisy Work with Retailers to Implement AI?
Sterling: Yeah. Well, that brings up a good question, because this AI, at least effective AI, can be so much better, and faster, and more accurate, and learn so much more than humans, a team of humans ever could. To bring it into a business, well, it starts to shake up some of the systems and processes they've got. I have to imagine, you know, if not replacing entire teams, like how do you work with retailers to take that journey to, you know, maybe baby step them into the world of retail tomorrow?
Gary: Yeah, that's a great question, Sterling. And I think that's the biggest challenge. I think all technology vendors have suffered this, they, they, they try to move the clients too fast, you know, too far too fast, right? And we certainly made that mistake in the early days. And, kind of, what we've learned is there's, there's really, I think, four phases of the AI journey in general that I would think. And it's about trust building, right? It's about building trust with the human users in retail, the merchants, and category managers, and the executives. And you have to bring them along the journey. And so, the first way we typically start that is, let's use AI to assess what they've, what the retailer's already done. So, the retailers built the plan, they execute it, AI can then come in and go, of these promotions, or these price points, or these assortments, here's the red, yellow, green, you know, the good, bad, the ugly. And then, not only just score the past, but say, here's why, this is why this promotion wasn’t good, it was the wrong time of the year, or it was the wrong item, or it was the wrong price point. So, in terms of the ordinary retail mechanics, let the AI share the learning. And so, once the human being sees those learnings, they think, oh, that makes sense. It's, you know, I get it, I understand it, it's not insane. There's some real practical, you know, you're like, you've got practical learning, then, then there's some trust. And then, so now stage two says, ok, now how do I use AI in a forward looking way? Now the retailer says, here's my plan, ok? The retailer still comes up with a plan. Now the AI scores the plan before it's executed, red, yellow, green again. And then gives alternative choices when, when, when, when it says, hey, that's a red idea. So, this, it's the wrong product, the wrong price point, the wrong season, again, the same learnings as the other one, but now doing it in a forward looking way. So, the retailer can now take advantage of that and get the benefits. And now, when you get that level of trust, now, then the merchants go, oh, this actually works, I'm seeing the improved results. Then the third stage is, ok, now let the AI come up with the plan. Now, now that we've got this trust along the way, the AI comes up with the plan and the human beings evaluate the plan and say, oh, here's these exceptions, I would make these changes, you know, that decision is not consistent with my brand, or, you know, I got a better vendor deal, or there was a drought in California, I can't get my grapes. You know, and so, the human now is evaluating the, the plan. And then the fourth step is, once you've got trust there, that the AI created plans make sense, then you can just integrate the decision making into the back-end systems. And the AI plans it, executes it, and there's appropriate governance put in place that flags exceptions for the people. And we see that as the journey. We typically started at step three, like a technology vendor, kind of, you know, bit of head in the clouds, right? And we've learned that you got to go to step one, right? And meet the customer where they live, and bring them along, build that trust. And so, long winded answer, you know, that, that's what we've learned in doing this, you know, 30, 40 times with, with many customers.
How Does Daisy Define ROI?
Kevin: How do you define ROI?
Gary: It's, for us, it's total company. You know, we think, you know, so that's another defining element of AI, we're running the company. Like I said, the car, the autonomous car, this is like an autonomous retail. So, you know, we want to measure total company net income, that, that's the ultimate metric, you know. It's, you know, part of that is customer sat, you know, you want to be satisfying the retail customers, the retailer's consumer customers, and that will drive net income growth. And so, we say, year over year net income growth that we can attribute to all the detailed decisions that the AI makes. And that's what we deliver our customers, show them what, what portion of improved decision making was due to the AI, and then measure net, you know, net income ROI.
Does AI Enable Retailers to See Beyond Their Business?
Kevin: Does that mean, that in essence, it sounds like that AI has the ability to help retailers, sort of, see beyond the silos that, that very often that they, that they view a business in. They, where they're not, they're seeing things within their own particular vacuums. It sounds like AI has the ability to help them see beyond that and see the connectivity. And that even if this department maybe is making a little bit less money than that department, that department is making more money because of something in the other department. I mean, is that, is it able to, kind of, help them make those determinations?
Gary: Yeah, absolutely. When I talked earlier about the example of like, you know, the autonomous car with the laws of physics, well, we've created the laws of retail. And so, the laws of retail are some of these effects. So, we've learned that consumers don't buy products, they buy solutions, right? And so, that's one thing. Items have a halo, right? So, if you see, I always use this ground beef example, if you see ground beef on sale, a consumer sees that, one consumer will say I'll make a pasta dinner, and they go buy pasta, tomato sauce, bread, cheese, produce for salad. And another customer will say, oh, I want to make hamburgers, so they'll buy hamburger buns, condiments, produce. But every customer who sees that ground beef, you don't consume raw ground beef, you need to buy something else, you need other products to satisfy that use case. If you contrast that to like a case of water, you know, there's not a lot of use cases for water, you buy the case of water, you drink it, you don't need to buy three or four other items. And so, that's one, one factor that goes into this theory of retail. Then you know that it's not all upside, that there's a negative effect. Because the customer who was making hamburgers bought ground beef, you know, sausage sales and hotdog sales were displaced. Because there was hamburger buns in the halo of the ground beef, hot dog bun sales go down. Because it was on sale, I bought two or three-weeks supply of ground beef, and I froze it. And so, all those interactive effects are really what the AI can do. That's beyond human ability. If you have, if you have 100,000 products, just for a round number, you have 10,000,000,00 1st order halo effects. That's way beyond human ability, and that's what the computer can do. And that's, in contrast to, you know, retailers have done category management, you know, look at each category as a silo and run the business. And that's worked great. There's absolutely nothing wrong with that. I mean, there's a lot of successful companies. We're just saying, in this new world, that's post COVID, even, you know, more difficult, more competitive than ever, this is another opportunity to get, get smarter and look at those interaction effects, which is, which are mostly being ignored by category management, just because it's beyond human ability. But again, there's nothing wrong with what the retailers have done to date. They're massively successful. It's just a question of is the risk of the status quo, is that more or less than the risk of change? Right?
Has Daisy Changed as a Result of COVID?
Sterling: Let's go into that question about COVID for a second. I mean, I speak in, run workshops on innovation. So, by April, people were calling me like, oh, you've got this figured out already. And it’s like, well, we're on a path, but it's painful. Now, Gary, you're the CEO of an AI company. How have you adapted and shifted because of COVID? I mean, have you changed the company? Are customers asking for different things? Has the product evolved at all? What's been the response?
Gary: The pain points post COVID are different than pre COVID, right? So, I see the things, the topics that retailers are most interested in these days that we see are our forecasting, obviously, knowing what products are going to sell, how much, you know. We know that you've walked into your stores and seen some empty shelves during this, kind of, pandemic. And so, wanting to know what's next. Yeah, right? So, wanting to know what's next. That's a big concern, forecasting. Another one is assortment planning, right? So obviously, you know, we've seen narrowing of assortments to make sure that you can have, that you're, you know, confident in the products that you, are in your assortment so you can get them. So, those are two, kind of, new kind of pain points that we've been responding to post COVID. Our core technology hasn't changed because we adapt to the new patterns of COVID. Like I said, that historical data, we don't need, our technology, the reinforcement learning, doesn't need a big, long history of data, because we're not modeling the history. We would look at the new halo patterns, the new cannibalization, the new elasticity, the new price elasticity, promotional elasticity, those factors are captured in as little as one day of data. So, our system can adapt to the new patterns that occur one day after it's happened. I don't think anybody could have predicted the run on toilet paper, you know, and, but as soon as it happened, on day two, we should have been able to respond to that. And so, that's what our technology can do. So, we, so our technology is, kind of, is not taken that, you know, modeling history. If you're modeling history, I don't know what you're going to do next February, March, because last year will be so different than what's happening now. But taking into account those fundamental theoretical attributes is what our system does. And yeah, and we're just having different conversations with our customers. As always, we want to respond to our customer pain and that's what we continue to do.
How Can AI Make Decisions by Integrating Outside Data?
Kevin: So. let's drill down on that a little a little bit, though. For example, I've, every retailer I've talked to lately is, is trying to figure out Thanksgiving, right? They're trying to figure out, they got, you know, it's at the end of, end of November, and they're, and Christmas as well. Do they buy as many turkeys to sell as they have in past years? Do they buy smaller turkeys? They’re thinking that's probably the case. So, do we cut, do they cut here? And then what do they do about, you know, about the, some of the accompaniments and things like that. To what degree could AI help them make some of those decisions by integrating outside data?
Gary: Certainly, outside data is part of the story. So, looking at, you know, market share data, I think, and seeing what, see how the market is performing. I think the biggest thing AI can do is, is take the best guess as to the level of traffic that's going to come in the store. Once you know the traffic walking in the door of your stores, you can have a pretty good estimate of how much you're going to sell of every product. And then, and through the halo relationships, you know that if somebody buys a turkey, you know the 10 other things that are likely to be, most likely to be bought with that. So, I think what AI can do is make that best estimate, looking at the post COVID world, seeing how different that was than pre COVID, and then seeing, you know, that estimate of how many people are coming in the door. Because once you know the people in the door, you have a good, you know, we know what converts, you have lots of data about conversion. And we have that in the, in the COVID data as well, the conversion rate of traffic to customer. So, I think, I think that's where AI can give you a best guess. Will it be perfect? No. But I think AI can do, you know, can do a better job than just people alone.
Does Every Retailer Have to Have AI?
Sterling: I'm just gonna say retailers that had AI in place around their pricing were probably in a much better position through March and April, and maybe even going into Thanksgiving and the holidays here, than anybody else. Because they've got this learning that's learning faster than the pace of time, which is especially helpful if there's an anomaly like a pandemic. Can retailers compete with this, Gary? I guess my question is, if enough retailers start to implement AI for pricing, does it become a cost of entry? Does everybody have to have it?
Gary:
I believe so. I think it's a cost of doing business. And I hope that, you know, in some, in some way, you know, retailers have been slow to adopt technology. In some ways, they've led the curve in technology adoption. And in some ways, they've lagged. And I hope that this opportunity will show retailers that technology is a critical piece of their business and AI is a, is a, is game stakes. Because, you know, you can't tell the difference between a company that's doing AI and a company that's not. A great AI promotion is, is invisible, great pricing is invisible, great inventory allocation is invisible. You're just watching your sales erode and wondering why. And so, I think being efficient and intelligent is game stakes. And I think, I hope that retail really takes this as a rallying opportunity to, to reset, reset the way they think about their businesses. You know, you can reset once a decade, once every few decades, I think this is a moment for retail to say, now let's rethink about the way we do promotions. Let's rethink this. And I think AI can play a big role in that. But definitely game stakes I think going forward. And we'll see, my belief is that once the, the COVID tail winds, you know, kind of, stop in the grocery business, that we'll, we'll see some, if you're struggling before COVID, you'll be struggling when the world reaches some new norm.
What is the Cost of Implementing AI?
Kevin: Is it expensive? I have no sense of what a, what, what it costs for a retailer to get into AI.
Gary: I can speak to what our price points are. I mean, we, we, we will guarantee a 10 to 1 net margin return on investment. So, for every dollar you pay us, we want to see you make at least $10. The larger the company, that ratio gets out of control. I mean, our biggest customers who are, you know, 20, 30 billion-dollar companies, they're, they're getting, you know, 10,000 to 1 return on our fees. So, I mean, that's our, you know, my focus has always been net income impact. And so, we stand behind that. That, and as long as you're using the technology, and we've figured out how to work together, and we overcome that change challenge. Every single customer we've been at, we've seen the same type of metrics, the same possibilities, just a question of, you know, can we build a mutual trust? And then, for us, what, you know, we price it so that there's a guaranteed return. I think, you know, some of the bigger technology companies, their solutions, you know, could be expensive. They're I think out of range for mid-market companies. I think, for the biggest companies in the world, they can afford to buy the IBM's and the SAS’s, kind of, technology. But, you know, our price points fit everybody. Because we'll price it for, our smallest customer has four stores. And as, you know, 60 to 80 million-dollar retailer, our largest company has hundreds of stores. And as a, you know, 20, 30 billion-dollar retailer. And the technology works across the spectrum. And we’ll price it to guarantee the return on investment.
Where to Find Out More About Daisy
Kevin: Well, that seems like a pretty good place to stop. So, Gary, if people want to find out more about Daisy and get in touch with you, what's the best way to do it?
Gary: Yeah. You can look at our website daisyintelligence.com. You can also look at, look up my last name, you're likely only to find me, and my children, and my brother on LinkedIn. And so, my LinkedIn has all my personal contact info. So, either of those two ways.
Kevin: And that's, and that's, so they know how to spell it, it's, it's in the text, but it's also s-a-a-r-e-n-v-i-r-t-a. And I've had all sorts of trouble today just pronouncing it, so, you know, that's how to spell it. Well listen, thanks to Gary Saarenvirta for joining us today. And thanks to you, the audience, as always, for spending time with us. This Retail Tomorrow podcast is brought to you by accelerate, empowering both retailers and brands by using its national network to get products to consumers. For more information on how to grow your business, go to www.accelerate360.com. I'm Kevin Coupe.
Sterling: I'm Sterling Hawkins.
Kevin: And we'll see you next week. In the meantime, be safe and stay healthy.
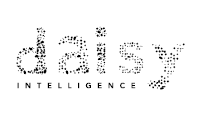