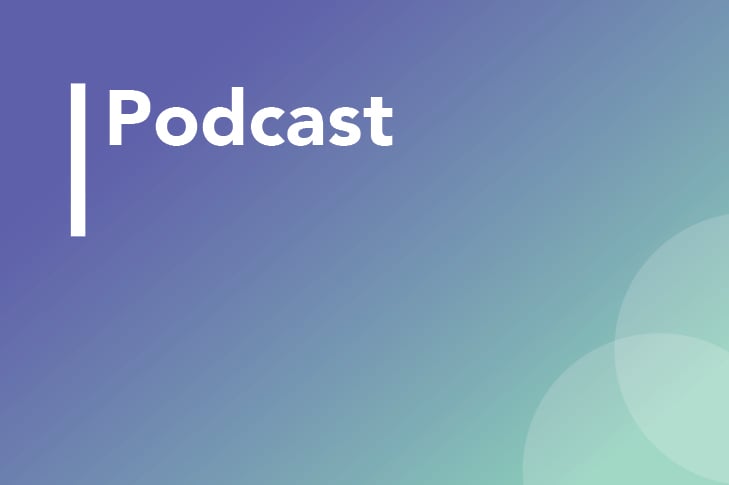
Gary Saarenvirta on the FraudPod Podcast [Video]
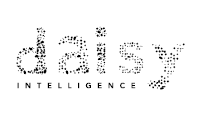
Watch The FraudPod Podcast
Daisy Founder & CEO Gary Saarenvirta joins Matthew Smith of The Coalition Against Insurance Fraud (CAIF) on the first episode of the FraudPod podcast to discuss automation in the insurance industry.
Original source: FraudPod Ep.1 from the CAIF on Vimeo.
The FraudPod Podcast Transcription
Matthew: I'm glad to be here today with one of our newest coalition members, and that is Daisy Intelligence. And with me today is Gary Saarenvirta. Gary is the CEO of Daisy Intelligence and is joining us today to talk about a number of issues. He also has a very interesting background. Gary's the former head of IBM Canada analytics and data warehousing practices. Now you'll hear from Gary directly, and he'll probably fulfill this for you, but he's a self-described tech evangelist and believes deeply in the societal benefits of machine learning technology. So, those are some of the things that we're going to talk about. But welcome, Gary, and it's great to have you with us today.
Gary: Well, thanks very much, Matthew. I look forward to chatting with you today.
How Did Daisy Get Started?
Matthew: Gary, one of the things that I always talk about is that we are in what I call the accidental profession. Nobody ever sets out to have a career in fighting insurance fraud. So, maybe for those joining us today, you could first tell us a little bit about your background, what led you to a career in insurance fraud investigation. And then also tell us about Daisy intelligence and how that got started.
Gary: Sure, I'd love to tell that story. So, you know, I have a background in aerospace engineering, I have a graduate degree from the University of Toronto. And when I graduated from school, you know, there's not a big aerospace industry in Canada, and I wasn't ready to move south of the border. And so, I started working in the corporate world and really found an opportunity to help bring more math and science to business, coming from a math and science background. And one of the careers I had was running that IBM's, kind of, analytics and data warehousing practice, as you mentioned, and I got exposed to fraud and risk solutions at IBM, and I was responsible for some of those solutions. And I thought that, you know, helping insurance companies minimize fraud would be a great societal benefit. I saw that some of the, some of the proceeds of fraud are used in very nefarious and terrible ways that impact society, and that funds, kind of, criminal activity. So, I thought that, you know, both it's a technical challenge which satisfied my, my technical background and my desire to apply my skills, and I thought also is a great societal benefit if we could eliminate fraud, and lower the cost of insurance, and eliminate some funding sources for some nefarious individuals who are doing bad things.
What is the Story Behind Daisy’s Name?
Matthew: Well, that sounds interesting. One thing that intrigued me, how do you make the bridge from the aerospace technology to the world of insurance fraud? And how did those initial meetings that you were in and concepts, kind of, develop to form Daisy Intelligence? And along with that, you also have to have some story behind how Daisy came to be in the name. So, maybe you could share that for us as well?
Gary: Yeah, sure. Sure. I'll start off with the Daisy naming story. I mean, so we named Daisy was, I wanted a name that was kind of like Apple, you know, that was, that was, kind of, very friendly. And so, our ad agency discovered that Daisy was the first song ever sung by computer in the late 60s. You know, ‘daisy, daisy, give me your answer, do’, that song from the 1800s. And so, the words ‘daisy, daisy, give me your answer, do’ was the first song sung by a computer. And then also, the flower, the daisy flower, the number of petals in the flower, is from the famous Fibonacci sequence. So, it was a very technical back, technical backstory to Daisy. And so, I thought that satisfied all things, it was, kind of, a friendly name and it has very scientific and really a numerical background. And so, I, that's how, that's how the name came about. And I'll talk about how we got, you know, I spent 25 years in the, in the commercial world that, you know, I started, got introduced to machine learning in the early 90s and doing predictive analytics. You know, that’s another term for really machine learning and AI. I think when most people say AI, they mean predictive analytics. And I was really astonished in the 90s how powerful that could be and then, kind of, along the way realized that machine learning alone, or predictive analytics alone, doesn't do the job. And I thought that what the aerospace industry has been doing for decades as well, you know, this recent Mars Perseverance lander, that landed on Mars, it was no human in the loop. It was an autonomous system. And so, doing, kind of, automatic decision making, you know, the aerospace industry has been doing that. And so, taking some of those methods, which engineers call optimal control, you know, they integrate the laws of physics and figure out what the right planning decisions are. And so, I thought that technology combined with the machine learning would help bring automation and decision making. And the predictive modeling alone has a few technical challenges, which I think, I think this, kind of, combination with the aerospace approach really, really brings to bear. And since that was my background, I found it was a unique way that we can default some of the challenges that are out there in the industry today.
Matthew: Well, you know, Gary, you may force me to correct those who have heard me speak about insurance fraud for years, in some of my presentations have heard me said, say that it's a fascinating profession, but it's not rocket science and we're not putting a man or woman on Mars. So, I think you actually corrected that. And maybe in the future of AI, there is, in fact, an equivalent of the aerospace, and putting people on Mars, and fighting fraud.
Gary: Yeah, I think, I mean, to be really, to be honest, I mean, being an investigator is a tough, tough job, you know, especially we have all this data now about claims, about claimants, about the third parties, providers, you know, all the individual, individuals involved, it's super complex. And so, you know, the people in the industry have done a fantastic job given the capabilities they had. And I think now, there's this technology available that can augment the human ability. And so, I think borrowing, you know, I think the aerospace industry and technology industries have created all kinds of spin off technology benefits. And I think that's what this is, you know, bringing some of those spin off ideas to really help the people. And our goal at Daisy is really we want to make the job easier, help insurance companies, and insurance investigators, and adjusters, make their jobs a little bit easier. And if we can help with technology, augment their expertise, and provide some contextual guidance, and maybe provide automation, where it's crystal clear and we don't need a person to spend their energy on, I think that's a great application of technology. That's really our mission at Daisy, really help make the job easier, you know. And if we can achieve that, then I know there's a financial outcome that, that will happen.
How Do Fraudsters See the Rise of AI and Are They Using Similar Technologies?
Matthew: Yeah, that's interesting that you would talk about that, Gary, because the coalition and our members, like Daisy, are SIU investigators, you know, all are on this side of using technology as a great tool to fight insurance fraud. From this perspective that you have, what are you seeing in terms of the fraudsters and the scammers? Kind of, look at it for a few moments through their eyes. How do they see the rise of this technology do you think? And perhaps even how are they using similar technologies to try to commit insurance fraud?
Gary: Yeah, that's a great question. I think, in speaking with some law enforcement people over the years in the space, I've heard that, you know, organized crime, disorganized law enforcement, and, you know, the organized criminals, they're very sophisticated. I've heard all kinds of, they build their own data centers, you know, they hire, hire 1000s of people, some to infiltrate within organizations. And so, they're very sophisticated. And so, I think they always look for the weakest link in the chain. And so, if one company starts to use technology and gets better at fraud detection, then those fraudsters move on. And I think it's, I think it's great that technology is starting to play a bigger role. And hopefully, we'll make it more and more difficult for those organized folks who are out there committing fraud. I think in the past, there's, there's a lot of loopholes. And because there's just not enough experts out there to go find all of that fraud. I think the bottleneck in the industry is having enough SIU people to really do the job. You know, it's hard, hard to find these great people. So, there's a lot of loopholes leftover. And I think as we start to close those loopholes with technology and then cover the spectrum with technology and SIU people, then hopefully the fraudsters will be forced to move on. I think we can, we can win the war against fraud in the coming decade, for sure, I believe.
Will AI Replace Humanity?
Matthew: You know, one of the comments that I've heard that I dearly love, as we look at the future of artificial intelligence in our profession, was a comment that another one of our members made, which was artificial intelligence will not replace humans, but humans who failed to use artificial intelligence will be replaced.
Gary: Absolutely. I 100% agree with that. I mean, it shouldn't be, as a tech company, the last thing I want to do is be known for replacing humanity and unemploying people. And computers aren't good at everything, you know, our mission at Daisy is really let people do what people are good at, and let technology do what technology is good at. And there's two different domains. Technology is great at crunching numbers and computing. And people are really good at ambiguity, and strategy, and seeing linkages where there's not obvious things. So, I believe really that augmenting the human capability with technology, I think the technology has to provide some contextual guidance to help the person, the investigator, do a better job. And then I think there's a big ocean of opportunity where we can let the technology just, we can automate it, and let the technology make the decision because I think there's a lot of low value high volume claims that the technology says that's a no brainer, I can just take that. Because we don't want to waste, you know talented SIU people's time looking at mundane things. And I think from the mundane, where we automate to some light human touch all the way to SIU expert, there definitely is a role for people and I believe always will be. I think that's how we cover the spectrum and solve some of the ethical dilemmas that using technology poses.
What Should Insurance Carriers be Doing to Implement AI in the Fight Against Insurance Fraud?
Matthew: Gary, one of the things that we get the coalition to try to do is, kind of, have a crystal ball and look into what the future is. And that's never easy in any situation, certainly not easy in the world of insurance fraud. But you and some of our people joining us today may be aware that last year, we pushed out in September, the largest study that had been done on the use of artificial intelligence in the world of insurance fraud investigations. And one of the things that came back that was interesting to us, to looking at the future, is just about half of our insurance carrier responders said that in the coming decade they plan to increase substantially their expenditure on artificial intelligence and using that. Well, as you look at that, from the standpoint of being a service provider, what do you think are the kinds of things that insurance carriers should be looking at, considering, to get what you were just talking about, the right balance of how to use technology, combine it with their teams, and integrate it into the future of fighting insurance fraud?
Gary: Yeah, I mean, I see the same kind of statistics that we've seen, you know, half, or even more, of insurance companies are planning to invest in AI and more technology, some already have and are well on the way. So, that's completely consistent with some of the studies that we've seen as well. And I think, I think as companies look to work with vendors, I think you need to find vendors who have a balanced view, that you can't be delusional and believe the technology is perfect, that I mentioned. You know, it's a, it's a tool that provides guidance to people and augments people. I think that's important, finding companies who can generate verifiable financial results, you know, I think that's important, elevate the role of the person, you know, that's our mission, elevate the people, deliver verifiable financial outcomes. Use the right technology, it's not only about predictive analytics, or bringing some other technology to bear. Having a journey, like AI is not a one and done. I signed up a vendor, it’s finished, right? It's a multiyear journey to get from where you are today to the future. So, figuring that journey out and working with a company that has a roadmap. And so, all of those things that are involved, we call that AI done right, AI done right is all of those things. And, and I think we, we've learned being, early days, an arrogant technology vendor who thought we had it all figured out. And very quickly we realized how naive we were working with experts in the industry to realize that, you know, there's a lot of expertise already out there and figuring out how to how to make the tough changes from today, and creating a path to tomorrow, and working out step by step, proving value every step of the way, making sure we bring the people along and educate the people as to what the technology is doing. Those are all super important considerations. And so, I think when you're talking to vendors, having somebody with a really sophisticated view of that kind of journey, practicality, conservatism, I think these are hallmarks of the insurance industry as well. And so, finding companies that have that same kind of mindset is important.
What is the Path Young People Should Take to Enter the Insurance Industry?
Matthew: So, I remember vividly the first insurance fraud case that I handled as a very young attorney down in Florida. And at that point, we considered high tech that we had self-correcting IBM electric typewriters, and some watching this today are old enough to remember that. But we considered that high tech. Of course, the world is different today. But, Gary, along with that, you know, we're also seeing the retirement of so many of the truly outstanding leaders in the world of insurance fraud. And we thank them, but one of the things that coalition is really looking at in the decade of the 2020s is who are going to be the future leaders in this profession. So, from where you sit at Daisy intelligence, looking at someone who is younger, we have academic students that follow the coalition, things such as that, people just starting out in the insurance profession or data analytics. What would you say to a young person that's looking at the possibility of going into insurance fraud as a career, to investigate it? Either from the standpoint of working for an insurance carrier or working for a company like yours, what would be the path that you would recommend that they follow to learn more about our profession?
Gary: I think it's, I think that's a great opportunity for young people. I think, I think young people are, you know, very open minded, and want to learn new things, and want to use technology. And I think one of the challenges in the past is taking people who are experts and convincing them that there's a better way. That's, that's, that's not an, that's sometimes a difficult sell. Because you're, you know, with, with years of experience, it's that, you know, you believe that what you're doing is valid and absolutely is the case. And learning new technology, sometimes, is a difficult thing. But I think young people who have this desire to use the latest and greatest. So, I think learning a little bit of technology is important, you know, getting a bit of analytical, you know, background, you don't have to be super deep technically, but having enough to understand how to interpret information. And then getting a strong business sense. And I think very quickly working with those people who are on the on the retirement path, we want to get as much knowledge out of those people as possible because you, you can't replace that. And I think one of the reasons to get started really early in AI is to have systems that capture the decisions that these experts are making to capture as much of that expertise as possible so that corporate knowledge is stored in the databases. And then if the AI can systematize that expertise, then we can teach that to the new people coming along, right? And I think it's important to get started quickly because there's, there's not replacing those 25 year, 30 year veterans. And, you know, there's a lot of nuance to fraud investigation that technology alone can't capture. So, I think working with those people and getting a bit of analytical and technology knowledge. So, I think for me, my career path has been straddling both of those worlds. And I found that's been really fruitful for me. And I would, I would tell young people to do that. Learn from everybody, learn from those experts who are in the field today, they’re a wealth of knowledge. And I think the most valuable time I spend is my customers teaching me how naïve I am at many times. And that's how we've evolved our technology quite a bit.
How Can We Eliminate Bias and Prejudice from AI Systems in Insurance?
Matthew: Gary, before we let you get back to your, your business day, I think we'd be remiss if we didn't bring up what we often hear about concerns, though, about artificial intelligence. And especially here in the United States, there's been a lot of discussion about systemic bias, potential programming that skews based upon improper data, or improper considerations, whether that's racial, whether that's economic, and the fear that artificial intelligence may open the door to those types of improprieties being part of the insurance markets. And of course, if it is, part of insurance fraud investigation. So, I would really love to hear your thoughts and your comments about how, in this world of using artificial intelligence, how are the, can the systems be either created, or supervised, or correctly monitored, to make sure that issues such as systemic bias, unnecessary prejudice, are not included in this future of artificial intelligence or minimized to the maximum extent we can?
Gary: Yeah, that's a great question. It's a great concern, something very valid and to be discussed. I think, I think when you're talking about bias, so we can't use a technology that only makes decisions based on the past outcomes, because those past outcomes are biased to the nature of your business, be that you've targeted certain, certain ethnic groups or economic groups. And so, using predictive analytics technology alone has a risk of, of creating some bias. And so, using other unsupervised technologies, and using that scientific approach, one of our scientific theorems is that if you are different than the norm, then there's a high likelihood that you may be committing fraud, or you're a risky individual, and so different than the norm that's how we say, whether you're in a certain ethnic group, can you find different than the norm within that peer group, or different than the norm within an economic group. So, you're not biasing any individual, you're able to find outliers who are different from the norm in whatever peer group you do. And that's a way, that's called, kind of, unsupervised learning where you don't have to have outcomes in the data. And so, that, I think that's one way to address bias kind of balancing unsupervised methods that do this peer analysis, as well as using historical outcomes. And then importantly as well is explainability. AI cannot be a black box. So, if you deny somebody a claim payment because you thought it was fraud, you have to be able to explain that and justify it, not saying my predictive model said that, you know, it had these coefficients from these variables and that's why you got denied. It has to be more explainable than that. And so, some of the technology Daisy uses is really clearly defining why your behavior was different than the norm. For example, as a podiatrist, let's say you're going to the doctor, as a podiatrist, in urban practice, in a hospital, your billing behavior was very different than all your peers who are in the same types of practice settings against the same age of patients. And so, the fact that you're very different than the average, and we can pin it down to these seven billing codes are very different in behavior compared to all your peers, and that's an outlier. And we can say, that's why we denied the claim, and then you give that person an opportunity to say, well, the reason for that is, you know, it could be that they're in a very specialized practice, or, or maybe they are committing fraud. And so, I think being able to get right down to the clear explanation of what happened. And giving that to a human being, having that human being, that's where the marriage of machine and people, you can't just let the machine go off alone, giving that guidance to the investigator so they can make an assessment to try to eliminate those biases, make sure the outcome is explainable. So, those are things that our technology focuses on. And that's part of AI done right is making sure we can explain it, justify it, and make sure that we're not biasing it against any historical outcomes that might have existed in the business before.
What Value do Organizations Like the Coalition Bring?
Matthew: Okay, one final question. And as I mentioned at the onset, we were thrilled with the coalition to welcome you and your team as a new member earlier this year in 2021. What value do you see organizations such as the coalition and the ability to connect with others in the profession? What can we do to help partner in this transition? What role do you see the coalition playing in the decade ahead as we make these monumental changes in the profession that you described today? How can we better partner?
Gary: Yeah, I think that, I think the coalition has a, has a very important role. I think, bringing together like-minded people who want to address the same problem, and that networking, it's, you know, it's always, you know, it's a very big world and making sure that we're connecting the individuals who want to make a difference in this, hosting discussions like ours today, and all the other things you do, the events and opportunities to meet and talk, I think that's, that's, I think that's the biggest value. And you identifying what are the key issues in the industry, bringing, bringing solutions, and answers, and discussions to bear on, on the, on the, on the changes in the industry. The world is changing faster and faster all the time. And you being at the center of a large group of people who care about that, you can bring all the different viewpoints, and give them a voice, and have a discussion, I think that's super important. And our value will be, we're excited to, once we get back to in person events, networking in person with some of the other players, learning from them, sharing our story, finding collaboration opportunities, and hearing what other people have to say about this space. And so, I think the coalition is an invaluable organization and I'm excited to be part of it, excited for Daisy and our team to participate going forward and looking forward to the virtual event that's coming up. And hopefully that in person event later in the year, I'm very excited to meet you, meet you in person, Matthew, and shake your hand one day. That's, that's something I can look forward to.
Matthew: Well, Gary, I look forward to that as well. And we'll have you, and everyone marked December 6th and 7th here in Washington, DC, for when we'll have our annual meeting. And hopefully all of us gathered together and welcome you and Daisy officially at that time as a member as well. But that is all the time that we have for today. So, I want to thank you, Gary. And for those that you have joined us today, thank you for taking time to join us as well. If you've enjoyed this, check out more information, please, at the coalition's website, it's really easy, it's insurancefraud.org. And then if you go to the tab on the home page that says newsroom, you'll scroll down and you'll see podcasts and videos. And you'll find this and other information there. So, please check out that resource at any time. Thank you again, Gary, for joining us today.
Gary: Thanks so much. If I can have one little plug, I'd love to say that we're publishing a whitepaper about some of the topics we discussed about today. And look forward to that. I'm sure we’ll, you'll help us promote that to the coalition group as well. So, you'll see something about it in the future and come to Daisy Intelligence website, daisyintelligence.com, and find out more. And happy to talk to anybody who's interested in finding out more or just chatting about the fraud in general. Thanks so much for having me today, Matthew, appreciate it.
Matthew: And we will definitely do that as well, Gary. Thanks, everyone.
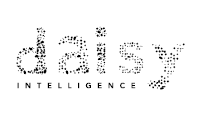